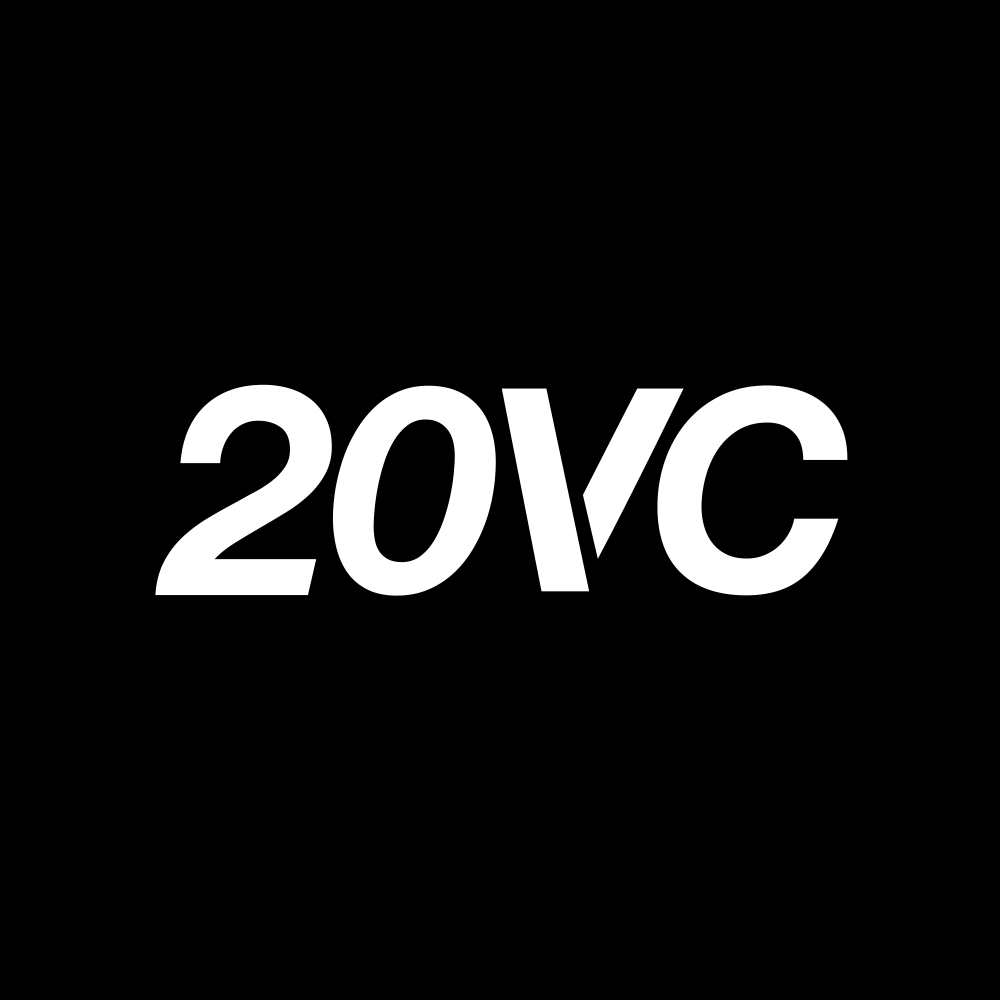
The Twenty Minute VC (20VC): Venture Capital | Startup Funding | The Pitch
20VC: Raising $500M To Compete in the Race for AGI | Will Scaling Laws Continue: Is Access to Compute Everything | Will Nvidia Continue To Dominate | The Biggest Bottlenecks in the Race for AGI with Eiso Kant, CTO @ Poolside
Mon, 07 Oct 2024
Eiso Kant is the Co-Founder and CTO of Poolside.ai, building next-generation AI for software engineering. Just last week, Poolside announced their $500M Series B valuing the company at $3BN. Prior to Poolside, Eiso founded Athenian, a data-enabled engineering platform. Before that, he built source{d} - the world’s first company dedicated to applying AI to code and software. 1. Raising $600M to Compete in the AGI Race: What is Poolside? How does Poolside differentiate from other general-purpose LLMs? How much of Poolside’s latest raise will be spent on compute? How does Eiso feel about large corporates being a large part of startup LLM provider’s funding rounds? Why did Poolside choose to only accept investment from Nvidia? Is $600M really enough to compete with the mega war chests of other LLMs? 2. The Big Questions in AI: Will scaling laws continue? Have we reached a stage of diminishing returns in model performance for LLMs? What is the biggest barrier to the continued improvement in model performance; data, algorithms or compute? To what extent will Nvidia’s Blackwell chip create a step function improvement in performance? What will OpenAI’s GPT5 need to have to be a gamechanger once again? 3. Compute, Chips and Cash: Does Eiso agree with Larry Ellison; “you need $100BN to play the foundation model game”? What does Eiso believe is the minimum entry price? Will we see the continuing monopoly of Nvidia? How does Eiso expect the compute landscape to evolve? Why are Amazon and Google best placed when it comes to reducing cost through their own chip manufacturing? Does Eiso agree with David Cahn @ Sequoia, “you will never train a frontier model on the same data centre twice”? Can the speed of data centre establishment and development keep up with the speed of foundation model development? 4. WTF Happens to The Model Layer: OpenAI and Anthropic… Does Eiso agree we are seeing foundation models become commoditised? What would Eiso do if he were Sam Altman today? Is $6.6BN really enough for OpenAI to compete against Google, Meta etc…? OpenAI at $150BN, Anthropic at $40BN and X.ai at $24BN. Which would Eiso choose to buy and why?
Who has earned the right to be in the race to AGI? We're going to look back on this moment 10 years from now, just like we would look back to the moment of mobile, internet, and realize that that was the moment where the table got set. You do not want to look back on that moment and not have given it everything you've got because it's a race.
And the latest $500 million round translates to us being able to be an entrant into the race. We don't get the luxury of stumbling on the capabilities race or to go to marketplace.
This is 20VC with me, Harry Stebbings, and there could not be a better time for this episode. Just last week, Poolside announced their Series B, a $500 million round, valuing the company at $3 billion. Today, we're joined by their co-founder and CEO, Iso Cant. This is an incredible episode on the future of LLMs, the race for AGI, how the chip and compute layer evolves, and so much more.
But before we dive in, this episode is presented by Brex. the financial stack founders can bank on. Brex knows that nearly 40% of startups fail because they run out of cash, so they built a banking experience that takes every dollar further. It's a stark difference from traditional banking options that leave your cash sitting idle while chipping away at it with fees.
To help you protect your cash and extend your runway, Brex combined the best things about checking, treasury and FDIC insurance in one powerhouse account. You can send and receive money worldwide at lightning speed. You can get 20x the standard FDIC protection through program banks. And you can earn industry-leading yield from your first dollar while still being able to access your funds anytime.
Brex is a top choice for startups. In fact, it's used by one in every three startups in the U.S., To join them, visit brex.com slash startups. And finally, let's talk about Squarespace. Squarespace is the all-in-one website platform for entrepreneurs to stand out and succeed online.
Whether you're just starting out or managing a growing brand, Squarespace makes it easy to create a beautiful website, engage with your audience, and sell anything from products to content, all in one place, all on your terms. What's blown me away is the Squarespace Blueprint AI and SEO tools.
It's like crafting your site with a guided system, ensuring it not only reflects your unique style, but also ranks well on search engines. Plus, their flexible payment options cater to every customer's needs, making transactions smooth and hassle-free. And the Squarespace AI? It's a content wizard helping you whip up text that truly resonates with your brand voice.
So if you're ready to get started, head to squarespace.com for a free trial. And when you're ready to launch, go to squarespace.com slash 20VC to save 10% off your first purchase of a website or domain. And finally, before we dive into the show, I want to recommend a book, The Road to Reinvention, a New York Times bestseller on mastering change. No time to read?
Listen to 20VC now, then download the Blinkist app to fit key reads into your schedule with Blinkist. you can grasp the core ideas of over 7,500 non-fiction books and podcasts in just 15 minutes, covering psychology, marketing, business, and more. It's no surprise 82% of Blinkist users see themselves as self-optimizers, and 65% say it's essential for business and career growth.
Speaking of business, Blinkist is a trusted L&D partner for industry leaders like Amazon, Hyundai and KPMG UK, empowering over 32 million users since 2012. As a 20VC listener, you can enjoy an exclusive 25% discount on Blinkist. That's B-L-I-N-K-I-S-T. Just visit Blinkist.com to claim your discount and transform the way you learn. You have now arrived at your destination.
So, dude, I am so excited for this. This is also the first time that we've actually met in person. You are far more incredibly good looking in person. So thank you so much for joining me today.
Well, thank you, Harry. It's a pleasure to be here. And it's glad that we finally met in person. It's been a minute since we've known each other.
Now, I want to just dive straight in. I think there's a lot of people looking at Poolside in the news and seeing the new round going, what is Poolside? Can you just provide some context? What is Poolside?
What do you do? Poolside's in the race towards AGI. We think the future is going to play out, that the gap between machine intelligence and human level capabilities is going to continue to decrease. But the path towards that, in our opinion, is by focusing on building the most capable AI for software development.
And all of this comes back to a set of foundational beliefs that we have that I would say are different than some of the other companies in the space in terms of where both research is heading and where capabilities are heading. The term AGI is a loaded term.
And the way that I like to kind of take the definition that is most commonly used is that at some point we are going to be in a world where across all sets of capabilities that we have as human beings, machine intelligence is going to be as capable and if not more capable than us and surpass us.
Now, our point of view is that that world is still quite a bit out and that we are actually going to end up in a place before that where we see human level capabilities in areas that are massively economically valuable and can drive abundance in the world for all of us that are not going to be equally distributed, not for every single thing.
And what I mean by that is that if you think about foundation models today, and I have a kind of simple mental model about them, which is that we are taking large web skill data and we're compressing it into a neural net and we're forcing generalization and learning. And this has led to things like incredible language understanding in these models.
But it's also led to things where we look at and we say, these models are kind of dumb. Why aren't they able to do X, Y, or Z? And our point of view is that the reason why they're not able to do X, Y, or Z has to do with how they learn. The most important part, I think, of what I said is the scale of data. When we have web-scale data, we can get language understanding.
But when we have areas where we have very little data, models really struggle to learn truly more capable areas. And I mean improvements in reasoning, improvements in planning capabilities, improvement in deep understanding of things. While as humans we don't require so much data, the way to think about models is that they require magnitudes order more data to learn the same thing.
Our focus is on software development and coding, and it's for a very specific reason. The world has already generated an incredibly large data set of code. To put a little bit into context, like usable code for training, what we refer to as about 3 trillion tokens.
And if you look at kind of usable language in English on the internet for training, we're talking about anywhere between 10 and 15 trillion tokens. There's a massive amount of code that the world has developed. Over 400 million code bases are publicly on the internet. So why don't we have this incredible AI that's able to already do everything in coding?
It's because coding is not just about the output of the work. The code that we have online represents the final product, but it doesn't represent all of the thinking and actions that we took to get there. And that's the missing data set. The missing data set in the world to go from where models are today to being as capable as humans at building software
is the data set that represents being given the task, all of your intermediate reasoning and thinking, the steps that you do, the code that you write and try to run, and then it fills and you learn from those interactions, and all the way to kind of getting that final product. And that intermediate data set, that's what Poolside exists on creating.
I immediately think, and you may chafe at this, but I immediately think of the social network where they are drawing the algebraic equations on the windows and you see that in the early scenes. How do you capture that process iteration thinking in what is previously non-existent or non-captured data?
This is the right question. The way I think about the world is that there are problems that we cannot simulate. The real world is impossible to perfectly simulate. It's messy. It's multivariable. How do we deal with the real world when we're trying to close the gap between human capabilities and AI? We have to gather data. The best example of this is Elon and Tesla.
Elon has put millions of cars on the road that are actually capturing every single engagement and disengagement with autopilot and every single scenario and extending that back to Tesla to train increasingly more capable AI.
And if you look at how full self-driving got more capable over the years, it's directly relational to it becoming more and more learning from the data instead of rule-based and more and more cars on the road. And so to me, Elon has won full self-driving. It's inevitable that
The most capable AI for full self-driving is coming out of Tesla because they've been gathering and building up this data set. And he needs to gather data because it's non-simulatable. Now, this is the head fake behind Poolside. You think about AlphaGo being deterministic. You think about the other end, the real world being non-deterministic. Where does code sit?
Code sits a lot closer to being deterministic. Follows a set of rules. Every time it runs, it runs in exactly the same way. And so this is what we call execution feedback. What we're really known for is our work in reinforcement learning from code execution feedback.
It's the way where we then take a model that we've trained from the ground up, we put it in an environment, say it's an environment with 130,000 real world code bases, several orders of magnitude, the largest environment in the world, And we sent the model off to explore different solutions to sets of tasks and learn from when it passes the tests versus when it doesn't.
There's a lot more details behind this, but the way to think about it is if you can simulate it, you can actually build an extremely large data set. And part of the things that we synthetically generate is not just the output code, but it's the intermediate thinking and reasoning to get to that output code.
Because models today, and you can try this yourself by going online and chatting to any model, can actually produce their thinking. They're not very good at it yet. So what do you do when your thinking is not very good? You need feedback. In our case, deterministic feedback, code execution feedback.
A lot of people break it down as compute, data, and then algorithms really. So if we take those three, how do you think about what the biggest bottleneck is today in the progression of models? Is it the data that we mentioned or is it one of the other two?
We are making in our space, especially I think post the ChatGPT moment, like incredible advancements in the algorithms that are making learning more efficient. Internally, I have this thing that I say to the team, and they're probably tired of me hearing because I say it every single day.
I say, all the work we do on foundation models, on one hand, is improving their compute efficiency for training or running them, or on the other hand, improving data. Now, the way to think about the algorithms and the improvement of compute efficiencies, that's table stakes. All of us, OpenAI, Anthropy, Google, et cetera, are doing this, and we're just constantly improving here.
And it's engineering and research combined. But the real differentiation between two models is the data. compute matters tremendously for data. Because if you think about poolside, and we spoke about how do we get this data, and I mentioned the word synthetic, it means that we're generating it.
It means that we're using models to generate data, to then actually use models to evaluate it, to then run it. And so compute usually matters on the side of the generation of data. But once we have all of this data, where we started today, we spoke about neural nets essentially being compression of data that forces and generalizes learning. Now, when we have small models,
We are taking huge amounts of data and we're forcing this generalization of learning to happen in a very small space. And this is why we essentially see these difference in capabilities. Larger models require, essentially, it's easier for them to generalize because we're not forcing so much data into such a small compression space.
And so my personal mental model of this is that the scale of your models, this has been shown over and over again, by the way, we owe a depth of gratitude to Google, to OpenAI, proving out the scaling laws, which essentially say as we provide more data and more parameters, more skill, hence more compute for these models, we get more and more capable models.
Now there is a limit to that most likely. If you think about it as the analogy to compression, your image that you had, you know, at high resolution compressed down to small resolution. The small models are the small resolution. We have generalization, but you're losing things. But in the infinite extreme, an infinitely large model wouldn't be doing any compression.
So there is definitely a limit at some point to model size. But what underpins all of this, to directly answer your question, is the compute. And the compute really, really matters. Your own proprietary advantages in your applied research to get great data or to gather it matter equally as much. But if you don't have the compute, you're not in the race.
I want to kind of unpack that kind of one by one. If we start, I mentioned the algos, I mentioned the data, I mentioned the compute. You said about kind of algos and how it approves model efficiency. Is there a limit to how efficient models can and will get? And does that kind of plateau at some point?
We are horribly inefficient at learning today. If you think about what drives efficiency of learning, it's the algorithms and it's the hardware itself. We've got probably decades, if not hundreds of years of improvements still left there and different forms of it over time. If we look very practically in the coming years, we are going to see increasing advantages on the hardware.
I'm going to see increasing advantages on the algorithms. But I hope everyone takes away that this is table stakes. This is something that you have to do to be in this space and you have to be excellent at it. It's not what differentiates you, it's what allows you to keep up with everyone else.
On the synthetic data side, a lot of people use it as a catch-all for like, oh, we've got a data shortage problem, but don't worry, synthetic data is here to save us. To what extent is all synthetic data equally valuable or is it more valuable in certain industries versus others?
I think the biggest cognitive dissonance that people have around synthetic data is a model is generating data to then actually become smarter itself, right? It feels like a snake eating itself. There's something that doesn't make sense in it. Now, the way that you need to look at that is that there's actually another step in that loop.
There's something that determines if from all the data that the model generated in my domain and software development, I have a task in a code base and the model generates 100 different solutions. If I would just feed those hundred different solutions back to the model in its training, the model won't get smarter. That's the snake eating itself.
But if you have something that can determine an oracle of truth that can help say, this is better and this is worse, or this is correct and this is wrong, that's when you can actually use synthetic data.
But I do want to just discuss scaling laws before that. You mentioned it earlier. There's different opinions around this. A lot of people now have come to the conclusion that we haven't even touched the surface and scaling laws have so much more room to play out and others have a lot more negative views, bluntly.
How do you feel about where we are in terms of scaling laws and how much room we have to run?
We are starting to understand that the scaling, the first version of the scaling laws that came out spoke about the amount of data we provided during training and the size of the model. More data, longer training and size of the model larger requires more compute. And so we often say the scaling laws are about applying more compute. And it's actually more correct than we initially realized because
The importance of synthetic data for models to get better is another form of using compute, but we're using it at inference time. We're running these models to generate these 100 solutions, generate 1,000 or 100 or 50. I think we have a lot of room still for scaling up models. We can do this by scaling up data, and we can do this by scaling up the size of the model.
Now, our opinion is that there's a lot of room to scale the number of parameters and size of models still. But there's something that we don't really talk about in our industry as much. We're training extremely large models. And by the way, we until very recently weren't even capable of doing so because we didn't have the compute and the capital.
This is why our fundraise has been so important to us so that we can have the capital to scale up. But what everyone does is that extremely large models, we can run cost efficiently for our end users.
You have a multi-trillion parameter model that is what we often, you know, often architected as an MOE, meaning that not all of those parameters activate during inference time, but they're still very large. It's too expensive. Every request that you make to that model is not a couple of cents. And so you have to find a way to actually build models that you can actually run for customers.
And so what happens in our industry, and this is our path as well, is you train a very large model. where you can clearly see that there's more capabilities in the model. And then what we call, we distill it down to a smaller model.
And because this is the thing, learning from data models are really inefficient, but learning from data in combination with learning from a smarter, larger model is actually quite efficient. We make really big things that become really smart.
We then teach the smaller models to try to match as much of that intelligence as possible, which we can then economically viable, put in the market and make revenue from.
Just continue on that thread. We'll come back to the compute element. How do we expect the cost of models to change in the next 12 to 24 months?
We should separate the price and the cost of models. If you look at what's happening in the world of general purpose LLMs, LLMs for everything, it's an incredibly competitive price war that's happening. And it's happening between the large hyperscalers, and it's happening between kind of referred to as the escape velocity AI companies, anthropic and open AI.
And then you throw in the mix, the vendors that are putting up the open source models from Meta and such. And I often think about what sits in that stack of costs. Well, what sits in the stack of the cost is a server, a box, the networking around it, a data center, the chips, right, the GPUs, and then the energy that goes into that.
And everything after that is marginal cost or variable cost of the running of the models. So we have to think about who has the lowest cost profile in the space, right? Who has the cheapest first principles capex that they're doing to run these models?
Well, that's the people who have as much of that vertically integrated and who have as much of that infrastructure already online and brought into the world. And this really is the hyperscalers. This is Amazon in number one, Microsoft in number two, Google in number three. But there's something interesting about all of those.
Each of those at different moments in time understood that they couldn't be reliant on hardware built by NVIDIA or AMD by someone else that also built their own. Furtest along that has been Google with their TPUs. Things currently at their fifth generation. They started early on this and they've been improving it ever since.
Then you have Amazon who's been working on their Tranium and Inferentia chips, their Neuron cores for some time. And Amazon has an incredible background, by the way, in manufacturing chips. And this is something I don't think people take enough credit to because while Google has to work with Broadcom to be able to bring TPUs into the world,
Amazon is working with the fabs directly and they have an incredible skill, right? They've done an amazing job in the cloud and Microsoft still earlier in their own chip journey.
Now, I know this is again a preamble to your question and I'm sorry for bringing this there all the time, but I think it's an important thing to understand because when you are buying Nvidia hardware and you're putting it in a data center and you're working with an Oracle or you're working with whoever it is in the space or even a Google or an Amazon or Microsoft,
you are taking that margin of that chip, right, the H100, the H200, the Blackwell generation coming up, and that has to be baked into your costs. The way that I think about this is that at the extreme end of it, Amazon and Google and Microsoft, as well as they come out with their own silicon, have a lot more margin to play with. And now then it comes down to business decisions.
And right now, since this is a war, and it's a total, you know, like it's an incredible race that's happening. I often refer to it as a drunken bar fight that's happening in our industry. is that we are, you know, all these companies are massively incentivized to drop the cost of their models as quickly as possible. And they do that in two ways.
They do that by cutting more and more of their margin down to their actual cost, right, their hardware. And then you can see a big difference between, you know, what an Amazon is able to do and a Google and a Microsoft and OpenAI and Anthropic. But they also do it on the intelligence layer. We spoke earlier about large capable models that distill down into smaller models.
If you have the most intelligent largest model, you can distill it down into a smaller model and you can have advantages at that layer as well. My view is, though, that in the extreme of it, the compute margin, like the hardware margin, really matters as this gets lower and lower in price. And this is what we've seen in cloud computing as well.
Do you think in five years time we will need to go through the process of distilling a larger model down to a smaller model, trying to get the best of it for the benefits of reducing cost for end consumer or actually will have such efficient costing that actually it'll just be one model that we can apply?
This is my personal view of how the world plays out. It's really easy to stay focused on the tactics and things that matter in this moment. And they're exactly the right questions of what matter right now in the moment. But we are moving towards a place where we are closing this gap between human intelligence and machine intelligence. And I think it's going to be an incredible...
incredible amount of problems and challenges and places where we want to apply this intelligence. If I have a view on modern history, and my view on modern history is that if you look at what happened from the printing press onwards, is that what we've done is we've connected more and more people around intelligence.
We went from, you know, the telephone to personal computer, to the internet, to the mobile phone. Fundamentally, what we've been able to do is we've been able to take hard challenges in the world, that's cancer research, or if that's even building a business, a SaaS company, anything, and we've been able to connect more and more people together to direct resources to those things.
What we're fundamentally doing is we're bundling intelligence. More and more people got connected together, and I think that's the true underlying thing that has underpinned this technological exponential curve we're on. If you think back about 100 years ago or 50 years ago, you can truly see it's an exponential. I don't think we want to live in any other moment in time.
And the reason I mentioned this to your question is that I think we are now going to go from a world where human intelligence and the amount of humans we had was the entire bottleneck to now we are having machine intelligence.
And so we compare investments in energy and chips and compute together with humans and have an extreme explosion on this exponential of all of the places in the world where we want to direct it to. My point is that I think there is a huge amount of places where this is going to be valuable. We will figure out the compute efficiency along the way.
The hardware will get more efficient because that's capitalism. As the opportunity is big, we'll direct things to make it more efficient.
Speaking of where it's valuable, you said about closing the gap. And specifically with regards to code, and we chatted earlier about that with regards to other industries, I think we chatted about voice recognition as an alternative. How do you think about this element of closing the gap and how that correlates to where value is and maybe where it isn't?
The way I think about this is there's things in the world that today we consider economically valuable. And that ranges from scientific progress to very mundane things. Office buildings full of things that we look at today and say, why can't that be automated?
So if we take what's economically valuable, the next thing we need to ask ourselves, what's the gap between models today and human level capabilities? And how large is that gap? And in some cases, the gap is actually not that large anymore. We were talking earlier about speech recognition. Models today are pretty much there.
Maybe there's a tiny bit left to say, but we've closed that gap into an incredible amount. In other areas, the gap felt like it was going to be impossible to close, but we're making a lot of progress. Come back to full self-driving, if you've been in your latest Tesla FSD update, that gap feels getting closer and closer to be closed. Now, there's other areas where the gap is really large still.
I think software development, our domain, we think the gap is still very large, right? What models are able to do is they're massively useful assistants and they drive massive economic value because of that. But between a model working with a developer today, there's a huge, huge gap.
And we want to get to a world where developers can work with models that are as capable as them and potentially even one day more capable. Now, the reason I mentioned this is so we've got the human capability aspect, right? What's the gap that's there? How economically valuable is the domain?
Then there's a next area I think that you have to ask yourself is how easy is it going to be to close that gap? And that comes down to data. Where can we get extremely large scale web scale data to be able to close that gap in areas where the intelligence gap is really big? Because the bigger the gap in intelligence today, the more data that we need to close it.
If you kind of use this as a, where can we find the data size related to how large the gap is between human and machine intelligence, and how economically valuable it is in the real world already, I think the intersection between those is the places where companies like us get to exist.
My immediate thought jumps to GitHub. Are GitHub not the best place to do that?
GitHub today has this incredible data set, almost all of the code in the world. GitLab is a player, but only in the private side, right? What sits behind the accounts of developers. GitHub is massive in public code and it's massive in private code. But private code, no one's allowed to train on. Not us, not open AI. So all of us have access to the same public data and it's the output data.
And so there is an inherent advantage from a capabilities race perspective. Another thing that we frame in our company over and over again is there's a capabilities race in the world. And to your point earlier, we say there's four things that matter. Agree with you on the three, but I'm going to add one. Compute, it's data, it's proprietary applied research, the algorithms, and talent.
Talent is absolutely key in this industry. In the go-to-market race, it's talent first and foremost, but it's also product and distribution. And distribution, Microsoft definitely has an incredible positioning in the world.
Can I ask, in terms of the compute element that we didn't discuss, when we think about compute underpins all of this and a lot of the data challenges that we mentioned, is $600 million enough?
No. $600 million that we've raised till date and the latest $500 million round is translates to us being able to be an entrant into the race.
And what that means is that the 10,000 GPUs that we've now brought online this summer, that came from this capital, allow us to make incredible advancements in model capabilities because of our ability to take reinforcement learning from code execution feedback and generate extremely large amounts of data, and then train very large models with it.
It is enough for this moment in time, but over time, it won't be enough.
How much do you think you'll need?
It's a very good question. There are real physical, real world constraints behind this. We've seen crazy numbers thrown out in our industry of, you know, compute cluster sizes and things like that. But the world actually still needs time to catch up with the real ability to do so. Today, interconnecting more than 32,000 GPUs is extremely challenging.
We're starting to be able to possibly interconnect 100,000. But right now, a million GPU cluster, a 10 million GPU cluster for training of models has both true algorithmic things that we have to overcome to be able to do this, and also has actual physical limitations still in the world. So we're not living in a world right now where unlimited money can buy you unlimited advantages.
It's why we get to exist with 10,000 GPUs.
Does cash correlate to compute? And what I mean by that is, if you have cash, can you go to your store and say, I want this amount of compute, or is it more than that?
I think, again, it depends on how much cash and how much compute. About a year and a half ago when we started as a company, there was a true imbalance between supply and demand in the world that even as a frontier AI company starting, everyone wants you to win. NVIDIA is incentivized to hyperscale. Everyone is incentivized actually to make early stage companies succeed with compute.
It's a lot easier when you're an early stage AI company to get compute than it is when you're an enterprise because they understand this is where the future is heading towards. But even then, there was a real mismatch between demand and supply, and we had to do an incredible amount of work of understanding the market, building relationships, and having plan A to Z to get there.
In the last six months, the world has still a huge supply shortage, and we can see this. If you're an early-stage startup, there's lots of paths for you. If you're a frontier AI company, you need to make decisions about who do you partner with, who do you work with, how much do you do yourself? I'm making decisions today that will impact us on compute in 12 or 18 months from now.
It's very rare to be at early stage companies where you have to make decisions right now that impact you on physical infrastructure a year and a year and a half later. Have we seen that demand supply imbalance change? The world has still far more demand for GPU and GPU-like compute than that supply that's available.
Larry Ellison said on a stage recently, it will require $100 billion to enter the race. That is the entry price. Do you agree with that as an entry price?
If you want to become a hyperscaler that is able to put data centers all over the world with GPUs in it that are going to allow you to serve these models to everyone, an infrastructure player, that's probably it. And that's probably just a starting point.
If we look at the massive CapEx investments that all of the cloud companies are doing, they're far above $100 billion when you look at them over the course of a couple of years.
Now, in the race towards more and more capable AI, closing that gap between human intelligence and machine intelligence, I think we are all pushing the frontier more and more possible, and we're seeing how that gap closes as we're scaling up our models and scaling up our data. I don't think anyone has a definite answer of how many dollars is it going to take from here to there.
If we knew that, we knew the outcomes. We're all on the frontier of what's possible right now.
You very wisely called it a drunken bar fight. One of my friends who runs one of the hyperscalers the other day said it's like the Manhattan Project where kind of everyone's kind of actually trying to get out, but no one actually can. It's far too late and it's like chips are on the table and we've got to keep going. How far in are we, do you think?
Is this just the tip of the iceberg and there is a huge amount left to be spent by the incumbents? How do you see that?
i think we need to separate spend from getting the world's most possible capable ai by closing this gap getting to agi closing the gap between human intelligence and are they not the same thing they're not the same thing because if you look at these models as investments that we're making to get intelligence out on the other end that needs to be economically valuable to end users right with lots of layers and applications and things in between the model the creation of models is capex
The operating of them, the inference to running of them, is OPEX. But the OPEX to run them requires extremely large-scale physical footprint in the world. Very simple. If we would spend, you know, $100 making a model and it will ever return $2 or $3 in terms of value to the world, it makes no sense, right? The world will punish it. It won't exist.
The huge scale-out that has to happen in the world for AI to become something that can tackle all of our world's problems and can feed in all of, from our software to our daily lives, requires huge footprint to run these models. It requires massive inference. And that means it requires data centers all over the world, close to end users, latency matters. And that requires a massive build-out.
And I think this is one of the largest build-outs that we've seen in physical infrastructure since, you know, the last couple of decades in the cloud.
In terms of that kind of build-out of physical infrastructure, it was David Kahn, I'm trying to get exactly what he said, but he said essentially you will never train a frontier model on the same data center twice. You know, the evolution of models is now outpacing the development of data centers. Do you agree with him when you hear that?
We're in a world today where the amount of data centers that can hold and power and have enough energy to power increasingly magnitude order larges of clusters is a very small number. I think he's absolutely right in this sense.
Now, the data centers from two years ago versus the data centers in terms of size and power requirement that we're going to see in the next two years look radically different, not just because the scale of number of servers and nodes that we're interconnecting,
this is the difference between inference right for inference we don't need all of the machines to be connected to each other in the same place for training we need them all to be connected to each other in the same room in the same place and so that massively changes what a data center looks like i think the show has done so well because i ask questions that people think why do you need that for training and not for inference i think it's a good question
When we're scaling up the size of these models and we're training them on more and more data and we're using more and more compute for it, at every single step that we're taking in the learning, every set of samples of data that we show the model, we need them to communicate with each other and share what they've learned. across the optimization landscape.
And so this means that if I would, you know, have two data centers that sit far away from each other, the amount of information that they have to share with each other, all of the different servers, and we're talking here thousands and tens of thousands soon of servers, you know, would make it so slow that it wouldn't be economically viable to train these models.
Once I'm running a model, I'm using a lot less servers to run it. So think of it as having lots and lots of copies of the model during training over lots and lots of machines that every time they see data to learn, they need to communicate with each other to continue to improve in their learning.
Can I ask you, when we look at kind of the build-out and the chips required and the compute required, to what extent is it a continuing NVIDIA monopoly and to what extent is it a more even playing field?
The dynamic that exists in the world today, we all owe a depth of gratitude to NVIDIA. When I started in this space in 2016, we were stacking 1080 Ti chips in racks of servers at our office. And NVIDIA already back then understood that AI was going to be world-changing. And no other company, the exception of maybe Google, had that deep of a realization.
But Nvidia had massive conviction on this and continued to double down here and has made more and more incredible hardware. The company that fast followed on that was Google. That's why we're on the fifth generation of TPUs. And the company that fast followed on that was Amazon.
The reason I mentioned those three specifically is that they are all building extremely large volume of chips and constantly iterating on faster and better and better generations of chips for training and for inference. They're for me the three primary players in the race, just from sheer volume of what they're producing in the fabs versus what they're bringing online to end users.
Now, we have some other companies in this space, AMD, competitor to Nvidia, doesn't have their own cloud, is reliant on being in this competitive nature from a price perspective with Nvidia. And so their ramp up is entirely determined by the demand of the world wanting to use their chips.
The demand of the world for AI for a Google and an Amazon with their own silicon is not the demand for chips, it's the demand for AI.
The way I look at this is that we're going to be in a world where those three and possibly new entrants or possibly, you know, AMD maybe catching up, but I think really those three and Microsoft with their own silicon one day are going to be the driving force in this industry.
To what extent has innovation in the space been held up by everyone awaiting NVIDIA's new Blackwell? I have to say that I was quite happy Blackwell was delayed.
Why? Because I'm training on H200s. And so the compute that I brought online at the end of August, these 10,000 H200s, mean that the longer the next generation chips is delayed, it helps me in a competitive nature in the world. But also there's a lot of marketing around the next generation of chips. And again, we have to separate training and inference.
Pretty much what we've seen consistently with every two-year generation of training from NVIDIA is about a 2x performance increase. But training is about 2x every two years. On inference, though, I think there's a lot of hope on Blackwell because it looks like for inference, Blackwell might potentially unlock a much, much larger gain.
When Blackwell is released, are you forced, given the competitive nature of the landscape, to get Blackwell 2 and to spend hundreds of millions of dollars on Blackwell chips upgrading from H200s?
The way we think about this, and I think the way to think about it, is that these chips, when they come two times more efficient, The operations we're doing on them is still the same. It's matrix multiplications and additions and such. It's math that we're doing on these chips. The Blackwell generation for us from a training perspective doesn't unlock anything new.
It just means that we have to, we can do more with a certain set of chips. My H200s become less valuable in the world, but it does not necessarily mean I have to go upgrade to the next generation.
We mentioned Blackwell and what that will unlock. I think a lot of people have been waiting for GPT-5 for quite a long time. When you think about what GPT-5 needs to deliver, what does it need to deliver to be a step function change? And do you think it will?
GPT-5, what it won't deliver, isn't a question we're going to look back on in a decade from now.
in a decade from now we're going to look back to this moment and it's similar i think how we look back to the early days of the computer the early days of the internet the early days of google and others and realize that we didn't fully internalize yet how much the world is going to unlock in value and abundance we wrote this blog post when the fundraising announcement came out we said look probably in this century there's three mountains that humanity is going to climb agi is one of the mountains the other is energy and the other is space
And so I think as we're going to keep progressing, we're going to keep looking at the next mountain, and from the top of that mountain, we look back, and we're going to realize the ones before were exponentially smaller.
We mentioned, like, is $600 million enough for you? I'm being slightly unfair here, but I don't understand how $6 billion is enough for OpenAI. You mentioned them in the hyperscalers.
But when you look at what Zuck has said he'll spend, what Google has said they'll spend, and Larry Page saying that he's willing to go bust in the race to win, and then Larry Ellison, I don't understand how $6 billion is anywhere near enough.
If we come back to the ingredients of the capabilities race, compute, talent, data, proprietary applied research, what we are going to find is that for compute, dollars have a direct one-on-one effect. But when we look at data, when we look at proprietary applied research and we look at talent, it is not as straightforward as dollars in magic success out on the other end of it.
I think we've had lots of examples in technology history already where we have seen these giants that seemed unbeatable. IBM in the early days of the personal computer. And so if we live in a world where we could perfectly translate dollars to successful outcomes, whoever can put more there is going to win. In the race towards AGI, dollars are critical for compute.
And remember, there's still time constraints. There's real-world physical constraints of how large we can make these compute clusters for training.
And it's that time and physical constraint, the constraint of what the chip is able to do, what the networking is able to pass through, that allow companies like us to have time and to do things and build massive advantages on the data, on the talent, and on the proprietary applied research.
Is there such thing as proprietary knowledge in this market, given the incestuous nature of jumping between companies and the knowledge that moves with those people? Is there such thing as proprietary knowledge?
I think you're fair to say that a lot of knowledge moves around.
What do you make of large corporates funding these companies? And do you have corporates in Poolside?
If you look at our capital raise, our last $500 million round, you'll see that there's none of the big hyperscalers, Google, Microsoft, Amazon were part of the round. Was that deliberate? That was deliberate from us. Because I think right now there's a future we see ahead of us for the world. And right now we see a path towards that future that we can do as a standalone company.
And we have to acknowledge the fact that we're all in the same race. And so to me, you can make strategic decisions along the way where you decide to say, hey, we're partnering up together in one way or another, like you're referring to equity relationships. It wasn't something that we had to do at this point. And it's something that, frankly, we very consciously decided not to do right now.
There is one corporate that became part of our round, and that was very deliberate, was NVIDIA. And it's because we collaborate really closely with them. I think that the nature of large technology companies choosing to invest in frontier AI companies is, frankly, the game theory optimal thing for them to do.
Do you think we will continue to see the consolidation of smaller players like Inflash and like Adept, like Character, continue to get acquired by the large incumbents?
I think there's very few left to be acquired, to be very honest. Who is left?
Cohere?
Juan? Reka. Reka. R-E-K-A. Small but very capable team from what I can see from the outside.
Where are they?
I believe they're in Europe. Mistral. And I'm hard-pressed to think of more companies. I mean, XAI is very unlikely to be acquired, but they're a very capable player in this space. And I focus, of course, on work in large language models and work towards AGI. So I think there's very few companies that today are sufficiently far along and that are still left.
You can buy OpenAI at $156. You can buy, and actually quite a lot of your investors said this was a great question, which I agree with. So you can buy OpenAI at $156, Anthropic at $40, which is their suggested new round, or X.AI at $24. Which one do you buy and why? It is an unfair question, but it's a good question.
I would love to spend a day with the current leadership team of every single one of these and then make a decision. They each have inherent advantages to them. XAI has understood that compute mattered and built an incredible team there and did what no one really had done at that speed. They built 100,000 GPU, three 32K interconnected clusters in Tennessee.
In the span of months, XAI showed up with Elon's strength, the ability to build physical infrastructure incredibly fast in the world. OpenAI had the incredible ChatGPT moment and has built this incredible business around both ChatGPT and the usage of their APIs and is clearly ahead in revenue as others, like as publicly stated.
And then I think Anthropic has incredible, thoughtful researchers and a very rigorous approach in what they do, a very rigorous scientific approach in terms of moving things forward. And so while I can see strengths in all three of those, it would really be a day with the with my own money.
That's awesome. Luckily, it's not your own money. You're a venture investor for this. And so which one would you go for? I'm not a YOLO venture investor. What would you do if you were Sam today? You just raised 6 billion.
I think Sam and OpenAI have understood the importance of compute and have understood the importance of data. What I imagine that $6.6 billion is going towards is exactly those two things. Where from the outside, I think it is tricky to be Sam today. I think it's tricky to be Sam today because general purpose models that aim to be everything for everyone is an incredibly competitive market.
And you find yourself with incredible pressures from all sides. And you're building a platform and a consumer product at exactly the same time. And more so than that, you're building a consumer product that from the outside is seeming to be for everyone. And I think that's a really hard thing to do.
It's funny, there was a brilliant Elon Musk interview, I think it was with Rogan, and he says, like, a lot of people think they'd like to be me. Not that fun. That one stuck with me. You actually really hear the sadness in his voice.
It's one I think about a lot, to be very honest, and you're getting me even with it. It's probably one of the only things you could have said that would have got me a bit emotional, because I think about it a lot. I saw it many years ago, and I think I understand what he means very well. I was earlier today with a founder I really respect.
And we were funny enough talking a little bit about this, is that building what we're building, it's not a choice, it's an obsession. And you bring everything you've got to it. And we were talking about, hey, how do you deal with waking up at three in the morning and your mind doesn't stop racing?
Going back and forth and sharing each of our techniques and probably going back home tonight and trying them I think Elon is one of the most impressive examples of someone who has done this for such a prolonged amount of time at moments in time when the entire world refused to align around his view.
I think there's companies in the world that get built because they were at the right moment at the right time. And there's companies in the world that get built that shouldn't have the right to exist. Everything is against them. And I think Elon has done that not once. He's done it multiple times. And it brings me to another quote that I heard on an interview from him or about him was Peter Thiel.
And Peter Thiel said, when we all worked with Elon, we thought he was crazy. He would take so much risk. And then he went and started, you know, Tesla and SpaceX. And we thought he was even crazier. And if one of those two companies would have worked out, we would have said he'd gotten lucky. But both of those companies worked out and beyond and to extremes.
But there's something Elon understands about risk that the rest of us don't. And for me, that's the second quote that's been on my mind most of this year.
Another fantastic Thiel quote is when he compared actually kind of crypto and AI, he said that crypto specifically really embodied decentralization. And if that was the case, then AI would embody centralization.
When I was in high school in 2008, my very first startup was a virtual digital currency. My views on crypto have changed a lot over the years. The notion of decentralization and what it can mean for the world is incredible, are incredible ideals.
The problem I think that we've seen in crypto is a quote that I learned, it might have been my high school economics professor, and he said, bad money drives out good money. If you think about this in environments, when bad actors come in, it drives out the good actors because we want to be in environments with other good actors.
and i think the promise of crypto started from great actors and found itself due to you know the incentives of the ability to make money very quickly in lots of distorted ways to bring a lot of bad actors to it the bad actors have driven out a lot of the good actors over time that are still a true amazing idealist in that space now the thing in ai that we don't have that
We have a set of people around the world who all fundamentally might disagree on how to get there, but all see that in 10, 15 years, we're going to look back and realize we had this incredible shift in the world by being able to close the gap between machine and human intelligence. And so while that might
drive today some centralization because of the sheer amount of resources required that are scarce, right? Capital is the least scarce part of this, right? The talent is scarce. The proprietary, you know, applied points of view and research, those are scarce. I think that does lead itself to a small number of companies. I think we've seen that over and over in history.
We look at when the massive boom of automobiles, right? The hundreds of automobile companies that started and how many actually survived. We've had this over and over again. And so I don't think this is something new. What I would like to see is that it's not just Google, Amazon, and Microsoft.
It's going to be an open AI, an anthropic, a poolside, and a set of companies who are able to get that massive escape velocity needed to sit alongside those companies and build the next generational businesses.
You mentioned bad actors there, and it made me think of tourists. And when I thought of tourists, for some reason I thought of like, bluntly, and this sounds awful, but like people who are not in it for the long term or who are in it for a story.
And a lot of public company CEOs and large company CEOs, and this is not tourists or bad actors at all, but they have to tell an AI story and they have to show that they are spending money on AI and innovating in some way.
My question to you is when you, you know, you mentioned obviously the GTM team build out, when you think about the revenues that we're seeing today, are we well past the experimental budget phase? Are we into true deployment, true commitment? How do you see that from enterprise?
So I think it depends on the use case. There's lots of experimental use cases still, and there's use cases that are far past the experimental side. AI for software developers, I don't think anyone in the world anymore questions that software development moving forward is going to be, for the foreseeable future, a developer-led AI-assisted world and an increasingly AI-assisted world.
Which use case do you see that you least understand or think has long-term potential?
I think there are use cases that are commoditizing very quickly. Speech recognition, I think, is one of them. Image generation is one already where we're seeing more and more commoditization over time.
You've mentioned talent before being such a crucial part that we haven't really unpacked because we have discussed the models, the data, the compute. The talent perspective is one that you also have taken quite a different approach on. You know, you're a European-based company.
The big question that a lot of your investors said that we have to discuss is, why did you decide to keep this as a European-based company?
I want to set the record straight. We're an American company, and we've got incredible people from all the way from San Francisco to Israel. But a decision that we made early on, Jason and I, my co-founder and I, we were planning on building this company in the Bay Area. And we did the work in the first days of the company.
And the work was let's make a list of everyone we think from both that we knew and also like externally, like, you know, on research papers and GitHub repos that we think potentially could be great for us. And the list ended up with about 3,300 people. A lot of work done.
3,300 people that we saw ranged from having experience on distributed training, to GPU optimizations, to work on data, to reinforcement learning, experience with large language models. So the whole breadth of what it takes to build what we're building from a model perspective. In that list was a location column.
And as you can expect, the number one represented geo in that was the Bay Area, not even the United States, just purely the Bay Area. But what really was striking for us is that there was a huge part of that list that was not in the Bay Area. It was spread out across Europe and Israel, from the UK to Switzerland to Tel Aviv to Amsterdam, you know.
harris like all of these different places well we couldn't see a clear deep talent concentration in one place probably uk actually being the one with the largest talent concentration we didn't realize that it was probably worth spending some time talking to people there and so i went and had conversations and and we realized one thing we said there's incredibly capable people here who want to stay here geographically they don't want to move to the bay area to join some of the other companies in the space
But they're not finding massively ambitious, you know, young companies that have huge visions to join. And so we saw that as an advantage, right? To the four things in that, you know, capabilities race, we need to build unfair advantages for every single one of those. And so we said, great, let's build up talent, you know, here on this continent in Europe, as we will in the United States.
And frankly, I'm very grateful that we did.
How many people do you have in London?
London for us is about 15 people.
How many in Paris? Two. Sorry, maybe I'm not allowed to go there. Paris is meant to be the AI hub of Europe, no?
The way to think about it is where has talent historically been, even pre-ChatGP team moment, and talent in AI, and who helped build that talent in the space? The number one company we have to give credit to is DeepMind. DeepMind built an incredible talent base. They built it out of London. Meta did some work in building a very incredible talent base, and it did it between London and Paris.
But in terms of when you look at it from a numbers perspective and sheer size of people, Google separately and DeepMind as part of Google had made much larger investments. And then there's another talent pool that we do often talk about publicly that is just absolutely extraordinary, which is Yandex.
Yandex built an incredible company in Russia with some of the world's most capable researchers and engineers, many of which have since left Russia and have kind of become a diaspora all over Europe.
When we look at that talent, when we think about work ethic, it's one thing which Europe is often chastised for. In terms of work-life balance, how do you approach that and feel about implementing standards of work with teams?
There was a tweet, and if I recall correctly, it's from Aaron Levi from Box, early on in post-ChatGPT moment. And he wrote something along the lines of, if you feel like you're working extremely hard on reasonable hours as AI is now booming, you're probably right to do so.
Because it's in these first years, and I'm probably going beyond what the tweet said, but I think it's in these first years, it's where the table gets set. Who has earned the right to be in the race to AGI?
The way that I've always looked at this is my personal perspective, and so is my co-founder, and so is Margarita, has put us in a place where we're going to look back on this moment 10 years from now, just like we would look back to the moment of mobile, internet, and realize that that was the moment where the table got set.
And you do not want to look back on that moment and not have given it everything you've got. Because it's a race. And look, most startups are not racist. Most startups are against yourself. But AGI is a race. And so our view always has been the team that we build is a team that is deeply passionate to be in that race.
And frankly, when you decide to join a race and you're upfront about it, you decide to try to become the gold medalist in swimming, that means that there are sacrifices that come with that. You don't get to have it all.
and so that's something that we've been super open about with people from day zero it's on our first intro call we talk about like do you want to join a race and frankly i have found no shortage of people in europe that want to do that i think there's a there's a stereotype about europe but the fact of the matter is that people who want to join races and do truly their life's work they're built differently and you can find them all over the world in every single country you just got to do the work to find them
Chase Coleman had an interesting kind of stat. In the two years subsequent the founding of Netscape, 1% of the enterprise value of internet companies was created. 99% was in the chasm between that subsequent two years and now, meaning actually it is such a long process and so much is to come. Does that not go against the idea of it being a race and is now different?
You know, history doesn't repeat itself, it rhymes. I think it was Mark Twain. I think that might be the mistake that we're possibly making looking at the past. And the reason that is, is because we're on an exponential in terms of technological progress.
I think in 1996, with Netscape, if I'm getting the year right, there wasn't this amount of people and capital that understood what the future might look like in the next 10 years. And it took some time to get there. Now, I could be wrong about this.
Another thing that I could see as a possible avenue of why I tend to disagree is there's a big difference between what was required to be built in 1996 versus what's required to be built today. bring it all the way back and try to steel man the opposite side of the argument is maybe it's exactly that.
And that the next couple of years are about these massive capabilities that we're moving the world closer towards AGI. And then when you look at the following five or 10 years, it's true. The huge economic value that's going to come from that will of course surpass the economic value that we have. I think the economic value is going to continue to surpass on the exponential that we're on.
But what I don't agree with, the companies that are being built today will not have a set of companies amongst them that will become the giants of the future that have helped enable this.
I think the concern that I have is you will use a huge amount of dollars to get to a level of advancement in technology that will then be leveraged by other people to build incredibly valuable companies.
If we look at battery in particular, where there's kind of been unbelievable breakthroughs in battery technology by companies that you will never have heard of that got acquihired, went out of business and then were bought for their IP. And it's a case of actually it takes a huge amount of money to uncover new breakthroughs. And then those breakthroughs are taken by someone else.
So if we take the battery analogy, I would actually think a little bit about BYD. Started as a battery company. Today is the largest volume of electric car sold in the world. And I do think there is a lot to be said about deep vertical integration. Look at Poolside. We're building foundation models.
with a mission towards AGI, right now in the world focused on bringing more and more capabilities of AI to software development, building a truly N10 business. Because I agree with you that the value is not going to only accumulate at the model layer. It's going to accumulate all the way to the end user.
And so in our point of view, the way that we kind of, I think, get to avoid, you know, what the future plays out in your hypothetical scenario is just by truly doing it end to end. But I still actually look at this thinking that there will be more value built on top of us in the future than what we can possibly unlock only ourselves.
Last one, and then we'll do a quick fire. You mentioned BYD, unbelievable journey. Are China really two years behind the EU?
No, they're not. There's a couple of interesting things that might not be as obvious unless you're in our industry. The research that still gets published openly, that doesn't get held back, that is most interesting, is all coming out of China in vast spades and majorities, something that wouldn't necessarily be obvious. But if you think about the game theory optimal thing to do,
Because they're not on the forefront of the world scene of AI. Actually opening up some of that research is the game theory optional, you know, optimal thing to do to be able to continue to attract talent. Because that's really what opening up your research does, right? It attracts talent to you.
I think China is at an incredible level of capabilities and in no way could be discarded or thought of as years behind on AI or AGI progress. We're working on technologies that we can see have massive societal impact. I think it's really important to be good stewards of that technology and that progress. And I think part of that is acknowledging that what we know about is the technology.
What we know about is our users, our customers. But we should be careful in terms of trying to know what's best for the world and how to think about massive geopolitical conflicts and things like that. And so what I have said in the past is that the best thing that we can do is the West.
is to keep making it as attractive as possible for talent from china we consider as a competitor right on a very large skill to come to our countries the easier we make it for one of those four major ingredients in the capability race and frankly one of the most important ones to you know help us accelerate i think is probably the the most practical advice i can give
I'm going to do a quick fire round because I could talk to you all day. So I say a short statement, you give me your immediate thoughts. Does that sound okay? Let's do it. Let's try it. Okay. So what have you changed your mind on most in the last 12 months?
I think in the last 12 months, it's a continued realization of the importance of scale of data and not only computing. Do you regret not selling Source to GitHub? It was probably the dumbest financial decision of my life, considering it was an all-stock offer and GitHub sold to Microsoft, I think, less than a year later. And it would have 3x the price. Far higher than that.
But I'm really grateful I didn't.
Why?
I'm sitting here.
Would you have done Poolside if you had sold Source? With complete honesty.
I think the question is, what could I have been able to do continuing on Source's mission? Because Source's mission was the mission we're talking about today with Poolside. And back in 2016, there were very few people who believed it was ever possible for AI to write code. But no, I don't think there's really no regrets there. I wouldn't be sitting where I am today.
And I don't think I would have become the person that allows me to go build Poolside today. And frankly, I'm really grateful that that event did happen because that's how I met my co-founder. That's how I met Jason. He was the CTO at GitHub at the time, and it started this many-year conversation on what the progress in AI looks like and its applicability to software development.
What do you think is the biggest misconception of AI in the next 10 years?
That progress is going to halt. What would cause progress to halt? Global conflict that disrupts the supply chain of chips. That is fucking meaty for a quick fire round.
I really can't unpack that in 60 seconds. If you could have any board member in the world, who would it be?
Mark Zuckerberg.
Why?
i think we all should give a lot of credit to mark zuckerberg in terms of having built an incredible company with a lot of conviction on what the future would look like when most people didn't agree with him if you look at what he's done on ar and vr in the last decade right from buying oculus to where it is today when most of the world just wanted him to stop
That required an incredible ability to have conviction for a future that's going to massively change the world. To get to AGI requires an incredible conviction for a technology to change the world. He's one of the people who has done that and someone very different than the people I have currently on our board.
What's the worst thing that could happen for AI with regards to regulation?
Regulation that fundamentally halts progress for small companies. The reality of regulation in many cases is that it becomes an expensive bureaucratic overhead and that harms the most young startups. It doesn't harm companies that have raised massive amounts of capital.
What specific regulations should be taken away?
The world is finding a balance and the balance that I'd like to see that the world is, and I think it's moving towards it, is to regulate the end user application of AI. Just like we've regulated the end user applications of any type of technology before. It's not the database that does harm, you know, how it's used.
And so for me, I would love us for us to continue to hold companies massively accountable for the end use of their technology to users, to consumers. Less so trying to put limitations on how much compute you're allowed to train on. We're building tools that are closing this gap between human capabilities and machine intelligence. We are not building the Terminator.
So what do you think of DST's Yuri Milner? I really like Yuri. I got to know him through several occasions over the last year, but I never got to understand him until I read his book. Most people don't realize that Yuri has a book or a manifesto. You can find it online for free. And earlier you heard me reference to looking from one top of the mountain to the next.
And that's actually, it's a metaphor that I took from Yuri. And because I think what Yuri has stated and said is that the sheer importance of scientific progress, what he often refers to as humanity's story, right? We have this incredibly special thing on this tiny little blue marble in the universe that is so immensely large.
And I think he really embodied, you know, the notion of we cannot let that flame die out. To him and to Elon's credit, you know, sharing the fact that the world is that one of the ways of doing that is to become a spacefaring civilization.
his whole view is that progress that's happening towards agi helps us to be able to take this special thing that we have you know humanity and and spread it across the universe and there is people who say this and there's people who believe it and who who understand how special this is and what it can mean and yuri's one of those people but at the same time he's been an incredible capitalist
He's been an investor that, from what I can see from the outside, that understood that huge technology waves were happening all over the world in different places. And he was a truly global investor. What he saw was happening in the US, he would invest in India, he would invest in Indonesia and across Asia and in all of these different places.
And I think very few people from the investment landscape have taken strong conviction on what technology is going to bring in the next 10 years and then found a way to place bets all over the globe.
have people tried to buy poolside no comment boat living biggest pro biggest con so i think we have to put some context here several years ago me and my better half and our golden retriever we were living in a fancy apartment we decided to get an old sailing boat to fix up together we put this old sailing boat in the harbor ceiling was falling apart something we did together we were passionate about and we love being on the water i love being on open ocean in particular
At some point, we were spending more evenings and nights on the boat where the ceiling was falling apart than the fancy apartment. And we looked at each other and we said, why do we still have the fancy apartment? Now, we got a slightly nicer boat after that and home became a sailboat. And I've gotten this question from friends, never, I don't even know how you know this, by the way.
I've gotten a question from friends. For me, I think the same thing about living on a boat is exactly the same thing of wanting to move the world, you know, forward. And I think part of it is the drive of freedom, right? The freedom of options, the freedom of like, what can adventure in the future hold?
And then the simplicity of not, of just being with, you know, the person you love and your dog in a small space and you've got your good internet connection and you do your work. You know, all the other stuff doesn't matter. The watches, the cars, the houses, none of this stuff is ever going to be the things you think back on. It's the journey. And for me, a boat kind of allows for all of that.
It keeps life simple and it gets me, allows me to, you know, fully immerse myself in poolside. And I have to admit, since I started this company, I've been home less than a month. And so it's been a lot of travel, but it's the journey that matters. It's not the material stuff.
What specifically did you think mattered that no longer does matter? Stuff. Did you go through a phase of getting stuff?
I did. I was lucky. I went through it in my early 20s. I got a lot of stuff. And then I got rid of all of it. For me, the sheer realization is that it's the journey with the people. The outcomes that we want to see in the world, they're part of the obsession. If you can see a future that looks like one that ends up being this incredible future, you want to build it.
But that moment, once you reach that, which will always be an ever moving goal, is not the interesting moment. The interesting moment is every single day with the people. And I think for me, I just learned more and more over the years is that I love people and I love the people I work with on my team. I think in every single person, there's something incredible.
And if you cut all the stuff, you cut all the money and you pick the hardest, biggest thing you could possibly, you know, focus your life on and then do it with amazing people, you get to have this incredible experience.
Penultimate one, as investors, we write investment memos and there's always a section called premortem, which is projecting ahead of time a reason why a company won't work. If you were to write a premortem on Poolside, what is the number one reason why it wouldn't work?
We're in a race. If we stumble, we put our foot off the gas pedal, we lose. And I think in any race, there's 101 places you can stumble. We don't get the luxury of stumbling on the capabilities race or to go to market race. We have to be excellent in both. If we make a misstep on either of those, we can fall behind. And if we fall too much behind, we're no longer in the race and we don't matter.
What question, final one, what question are you not asked often or ever that you should be asked?
I'm surprised how I usually don't know what motivates you. And people ask about the business, they ask about the outcomes, they ask about the future you see. Very, very few people ask you about your why. And I think that's probably the most important question.
When we talked about earlier about looking at those three companies, my first question to all of them, you know, and I have asked some of them is the why.
you why do you want the eventual outcome that you are pursuing so vociferously why do you do what you do i think that says a lot about a person and i think and at the end of the day in any race in any ambitious endeavor it's the people that make it happen it's not the dollars in the account those are the resources and inputs that you need and it's the people and so for me the why is a really important question to ask people and then i think it goes back to the toyota's five wise why do you do what you do iso
I realized that I'm not calm or at peace when I'm not working on the hardest possible problem that lends to what I care about in the world.
Why?
Because my brain's never going to turn off. I'm always going to wake up at four in the morning. It's always going to be this constant going over and over of like what you care about. And when I've done that in the past or things that weren't the hardest possible
the most ambitious possible thing in terms of like what mattered in the world, the thing that mattered the most, I just didn't feel at ease. And now probably never worked. I've always worked hard, but I've never worked as hard as I have at Poolside. While it's intense and it's stressful, I feel at peace.
Dude, listen, I cannot thank you enough for doing this. I so appreciate the speed of doing it after the round. And this has been so much fun to do. Thank you, Harry.
I really appreciate it.
My word, I love doing that show. If you want to watch the full interview, you can find it on YouTube by searching for 20VC, where you can see ISO in the studio. It was a fantastic one. But before we leave you today, this episode is presented by Brex, the financial stack founders can bank on. Brex knows that nearly 40% of startups fail because they run out of cash.
So they built a banking experience that takes every dollar further. It's a stark difference from traditional banking options that leave your cash sitting idle while chipping away at it with fees. To help you protect your cash and extend your runway, Brex combined the best things about checking, treasury and FDIC insurance in one powerhouse account.
You can send and receive money worldwide at lightning speed. You can get 20x the standard FDIC protection through program banks. and you can earn industry-leading yield from your first dollar while still being able to access your funds anytime, Brex is a top choice for startups. In fact, it's used by one in every three startups in the US. To join them, visit brex.com slash startups.
And finally, let's talk about Squarespace. Squarespace is the all-in-one website platform for entrepreneurs to stand out and succeed online. Whether you're just starting out or managing a growing brand, Squarespace makes it easy to create a beautiful website, engage with your audience, and sell anything from products to content, all in one place, all on your terms.
What's blown me away is the Squarespace Blueprint AI and SEO tools. It's like crafting your site with a guided system. ensuring it not only reflects your unique style, but also ranks well on search engines. Plus, their flexible payment options cater to every customer's needs, making transactions smooth and hassle-free. And the Squarespace AI?
It's a content wizard helping you whip up text that truly resonates with your brand voice. So if you're ready to get started, head to squarespace.com for a free trial. And when you're ready to launch, go to squarespace.com slash 20VC to save 10% off your first purchase of a website or domain.
And finally, before we dive into the show, I want to recommend a book, The Road to Reinvention, a New York Times bestseller on mastering change. No time to read? Listen to 20VC now, then download the Blinkist app to fit key reads into your schedule. With Blinkist, you can grasp the core ideas of over 7,500 nonfiction books and podcasts.
in just 15 minutes covering psychology, marketing, business and more. It's no surprise 82% of Blinkist users see themselves as self-optimizers and 65% say it's essential for business and career growth. Speaking of business, Blinkist is a trusted L&D partner for industry leaders like Amazon, Hyundai and KPMG UK, empowering over 32 million users since 2012 as a 20VC listener.
you can enjoy an exclusive 25% discount on Blinkist. That's B-L-I-N-K-I-S-T. Just visit Blinkist.com slash 20VC to claim your discount and transform the way you learn. As always, I so appreciate all your support and stay tuned for an incredible episode on Wednesday with Jeff Wang at Sequoia, who runs their $10 billion hedge fund. An incredible show to come there.