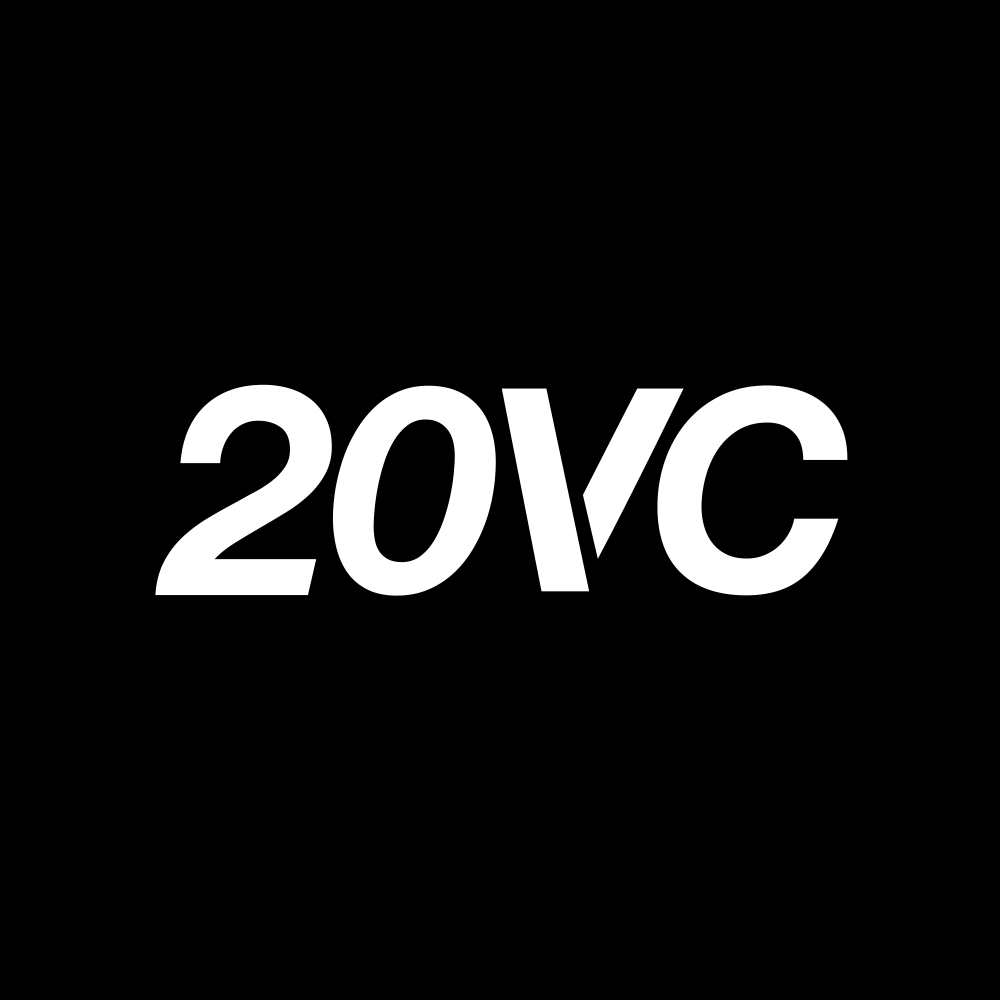
The Twenty Minute VC (20VC): Venture Capital | Startup Funding | The Pitch
20VC: OpenAI's Newest Board Member, Zico Colter on The Biggest Bottlenecks to the Performance of Foundation Models | The Biggest Questions and Concerns in AI Safety | How to Regulate an AI-Centric World
Wed, 04 Sep 2024
Zico Colter is a Professor and the Director of the Machine Learning Department at Carnegie Mellon University. His research spans several topics in AI and machine learning, including work in AI safety and robustness, LLM security, the impact of data on models, implicit models, and more. He also serves on the Board of OpenAI, as a Chief Expert for Bosch, and as Chief Technical Advisor to Gray Swan, a startup in the AI safety space. In Today's Episode with Zico Colter We Discuss: 1. Model Performance: What are the Bottlenecks: Data: To what extent have we leveraged all available data? How can we get more value from the data that we have to improve model performance? Compute: Have we reached a stage of diminishing returns where more data does not lead to an increased level of performance? Algorithms: What are the biggest problems with current algorithms? How will they change in the next 12 months to improve model performance? 2. Sam Altman, Sequoia and Frontier Models on Data Centres: Sam Altman: Does Zico agree with Sam Altman's statement that "compute will be the currency of the future?" Where is he right? Where is he wrong? David Cahn @ Sequoia: Does Zico agree with David's statement; "we will never train a frontier model on the same data centre twice?" 3. AI Safety: What People Think They Know But Do Not: What are people not concerned about today which is a massive concern with AI? What are people concerned about which is not a true concern for the future? Does Zico share Arvind Narayanan's concern, "the biggest danger is not that people will believe what they see, it is that they will not believe what they see"? Why does Zico believe the analogy of AI to nuclear weapons is wrong and inaccurate?
The real negative outcome is that people are not going to believe anything that they see anymore. Arguably, we are already well along this way where people basically don't believe anything that they read or that they see or anything else. It doesn't already conform to their current beliefs. It didn't even need AI to get there, but AI is absolutely an accelerant for this process.
It is a relatively new phenomenon that we have sort of a record of objective fact in the world. I mean, things like video didn't exist more than 100 years ago. Humans evolved at a time during an environment where all we could do was trust our close associates. That's how we believed things.
This is 20VC with me, Harry Stebbings, and today we are joined by OpenAI's newest board member and Carnegie Mellon's head of machine learning, Zico Coulter, from the bottlenecks in AI today to open versus closed systems to the biggest dangers of AI. This is an incredible discussion.
But before we dive in, when a promising startup files for an IPO or a venture capital firm loses its marquee partner, being the first to know gives you an advantage and time to plan your strategic response. Chances are the information reported it first.
The information is the trusted source for that important first look at actionable news across technology and finance, driving decisions with breaking stories, proprietary data tools and a spotlight on industry trends. With a subscription, you will join an elite community that includes leaders from the top VC firms, CEOs from Fortune 500 companies and esteemed banking and investment professionals.
In addition to must-read journalism in your inbox every day, you'll engage with fellow leaders in their active discussions or in person at exclusive events. Learn more and access a special offer for 20VC's listeners at www.theinformation.com slash deals slash 20VC. And speaking of incredible products that allows your team to do more, we need to talk about SecureFrame.
SecureFrame provides incredible levels of trust to your customers through automation. SecureFrame empowers businesses to build trust with customers by simplifying information security and compliance through AI and automation. Thousands of fast-growing businesses, including NASDAQ, AngelList, Doodle, and Coda trust SecureFrame
to expedite their compliance journey for global security and privacy standards such as SOC 2, ISO 2701, HIPAA, GDPR, and more. Backed by top-tier investors and corporations such as Google, Kleiner Perkins, the company is among the Forbes list of top 100 startup employers for 2023 and Business Insider's list of the 34 most promising AI startups of 2023. Learn more today at secureframe.com.
It really is a must. And finally, a company is nothing without its people, and so I want to talk about Cooley, the global law firm built around startups and venture capital. Since forming the first venture fund in Silicon Valley, Cooley has formed more venture capital funds than any other law firm in the world, with 60 plus years working with VCs.
They help VCs form and manage funds, make investments, and handle the myriad issues that arise through a fund's lifetime. We use them at 20 VC and have loved working with their teams in the US, London and Asia over the last few years.
So to learn more about the number one most active law firm representing VC-backed companies going public, head over to cooley.com and also cooleygo.com, Cooley's award-winning free legal resource for entrepreneurs. You have now arrived at your destination. Zico, I am so excited for this dude. I've been looking forward to this one for a while. So thank you so much for joining me today.
Great. Thanks. Wonderful to be here.
Now we're going to discuss some pretty meaty topics before we do dive in. Can you just give me the 60 second context on why you're so well versed to discuss them and your roles today?
Sure. Absolutely. So I seem to have be collecting jobs here. I have a number of different roles. I'm first and foremost, a professor and the head of the machine learning department. at Carnegie Mellon. I've been here for about 12 years. And here, the machine learning department is really kind of unique because it's a whole department just for machine learning.
And I've been heading it up actually, as of quite recently, and get to immerse myself in the business and the thought of machine learning all day, every day. Also, I am recently on the board of OpenAI, which I joined at this point a couple of weeks ago, and it's been extremely exciting as well.
Now, I want to start with some foundations and mechanics. When we look at kind of the basic techniques that underpin current AI systems, can you help me understand what are the basic techniques today behind current AI systems?
Right. So let's talk about AI as LLMs, but with, of course, the context that AI is a much, much broader topic than this. LLMs are amazing. The way they work at the most basic level, you take a lot of data from the internet, you train a model. And I know that's a very sort of colloquial term that we use here. But basically, what you do is you build a great big
set of kind of mathematical equations that will learn to predict the words in the sequence that is given to them. If you see the quick brown fox as your starting phrase of a sentence, it will predict the word jumped. We train a big model on predicting words on the internet.
And then when it comes time to actually speak with an AI system, all we do is we use that model to predict what's the next word in a response. This is, to put it bluntly, a little bit absurd that this works. So there's sort of two philosophies of thought here. People often use this sort of mechanism of how these models work as a way to dismiss them.
Oftentimes, I know people say, oh, well, AI is, it's just predicting words. That's all it's doing. Therefore, it can't be intelligent. It can't be. And I think that's just demonstrably wrong. What I think is amazing, though, is the scientific fact
that when you build a model like this, when you build a model that predicts words, and then just turn this model loose, have it predict words one after the other, and then chain them all together, what comes out of that process is intelligent. And I think it's demonstrably intelligent, right? I really believe these systems are intelligent, definitely. And I would say that this fact is
You can train word predictors and they produce intelligent, coherent, long form responses. This is one of the most notable, if not the most notable scientific discovery of the past 10, 20 years, maybe much longer than that, right? Maybe it was much deeper than that, in fact. And so this is not oftentimes given its due as a scientific discovery because it is a scientific discovery.
Can I just dive in and ask, you mentioned there the element of kind of the data input being so necessary. Everyone or a lot of people think that we've plundered the resources of data that we have already.
We will need synthetic data to really supplement the data that we already have, or we need to create new forms, be it the transcription of YouTube videos, which is like 150 billion hours or whatever that is. To what extent do you think it's true that we've plundered the data resources that we have available and we are running into a data shortage crisis?
two kind of answers to this question, which are diametrically opposed, as with many questions, right? Because you're exactly right. The thought is, because these models are built to basically predict text on the internet, if you run out of text, that would imply that they're kind of plateauing. I don't think this is actually true for several reasons, which I can get into.
But just from a raw standpoint of training these models, I mean, there's two ways in which this is sort of maybe true, maybe false. It is true that a lot of the easily available data, sort of the highest quality data that's out there on the internet has been consumed by these models. We have used this data. There is not another Wikipedia and things like this, right?
There's only so much really high quality, good text that's available out there. On the flip side, and this is the point I often make, first of all, we're only talking about text there. We're only talking about publicly available text.
If you start talking about internally available text, stuff like this, from a very straightforward standpoint, we have not gotten close to using all the data that's available. Public models that train on the order of 30 terabytes of data or something like this, right? So 30 terabytes of text data. This sounds like a lot, But this is a tiny, tiny amount of data.
And there is so much more data that's available that we are not using right now to build these models. And of course, I'm thinking about things like multimodal data, stuff like this video data, audio data, all these things, we have massive amounts available. I mean, just just a few tens of terabytes is not the amount of data these large companies that index the internet are storing.
There is so much more data than this, and we have not really come close to tapping that whole reserve. Now, whether or not we can use that data well, right, because text data in some sense is the most distilled form of a lot of this, and a lot of this is not textual data, that remains to be seen. But we are nowhere close to hitting the limits of available data in these models, right?
Arguably, we're unable to process it, because we don't have enough compute and things like this. But we're nowhere close to data limits in other senses. MARK MANDELBACHER- What are the challenges of using these new forms of multimodal data well? PAUL BAKAUSKI- I think the biggest challenge is simply compute.
If you have something like video data, just think about the size of a video file versus a text file. So if we transcribed this podcast, it would be a few kilobytes. If you take the dump of video from it, it'll be on the order of, I don't even know, I do. It would be about six and a half gigabytes. Gigabytes, exactly, right?
So tens of thousands of magnitudes of difference, orders of magnitude of difference, right? Now, arguably, depending on people's opinion, maybe the entirety of the actual valuable information is not in the audio of my voice and the video. You could argue that there's not as much usable content there. When we think about what kind of data humans use, I would argue that visual data
sort of spatio-temporal data, this is hugely important to our conception of intelligence, right? This is hugely important to the way that we interact with the world, the way that we sort of think about our own intelligence. And so I can't fathom that there is not a value to many, many more modalities of data, be it video, be it audio, be it
other time series and things like this that we sort of don't quite, that are not audio, but the sort of other sensory signals, stuff like this. There are massive amounts of data available. And I think we have not yet figured out how to properly leverage those due to either limitations of compute.
I mean, you have to process all that data and it does take, we don't have current models to do this very well, or just to do the limitations and sort of how we transfer and generalize across these modalities here. I think there has to be a use for it.
If we just took it to a logical extreme, though, and said we had plundered the reserves of data, and you mentioned that even if we had, we were not seeing a plateauing in performance of models. Why is that? Because one would assume so.
There are a few different sort of notions here. One is just the fact that we still seem to be in a world where you can increase model size and get better performance, even with the same data. So obviously, the real value of bigger models is they can suck up more data. They're able to ingest more and more data.
But it is also true that if you just take a fixed data set and run over it multiple times, if you use a bigger model, it will often work better. We have not really reached the plateau there.
The other thing, though, I don't think anyone would argue, or most people would not argue, that the current models in some sense extract the maximum information possible out of the data that is presented to them. And a very simple example of this is if you train a classifier, just to classify images of cats versus dogs on a bunch of images, you get a certain level of performance.
If you train a generative model on those exact same images, generate more synthetic data from that generative model, and then train on that more synthetic data, you don't do that much better, but you do a little bit better. And that's just wild. What that means is our current algorithms, we are not yet maximally extracting the information from data we have.
And there are way more deductions and inferences and other processes that we can apply to our current data to provide more value. And as models get bigger and better, they arguably can kind of do this themselves, either through synthetic data or through different mechanisms by which we train these models.
When we think about optimizing the data that we have in terms of kind of value extraction, what could be done further to get further value from the data that we have?
I don't really know, to be honest. I think this is a major open question right now in research. How do we extract the maximal information content from the data that we have? But again, as I said, I don't think we're close to even extracting all the data that's available.
When I look at this landscape, and we know that we aren't close to extracting the maximal information in the closure set of all the data that we have available... And we have not come close to processing all the data that's available to us. The idea that somehow this is a recipe for models plateauing in performance just doesn't jive to me with the reality of what we see.
So we're in the classroom together. We've got a big cross on that, like data, not the bottleneck. Good. Models, the joys of this show is I can kind of just regurgitate statements that others might have said and test them. Everyone talks to me about kind of moving to this world of many smaller models, which are maybe more efficient. To what extent do we agree with that? Is that right?
How should we interpret that?
We have not yet reached an equilibrium point where we have a good sense of sort of what the steady state of model size and for what application and how it's being used and is it being used as a general purpose system or for a very specific reason. This is all still being figured out right now. What I will say is that I use these models very regularly for my daily work.
I work almost exclusively with the largest models that are available to me because it just works better. And when I don't have a given task that I'm doing over and over, when I want to have that generality, I want to work with the larger models that are available.
The notion of sort of small language models and this kind of stuff, and again, I think this might be very much a possibility in the future. It kind of comes after we reach this point of generality, right? So once we've done something enough and we realize, okay, there is still a small task we want to do many, many times, maybe before we would have used...
custom-trained machine learning model for this. But the idea is that once you have a task, a rote task that you're repeating again and again enough times, and you know a small model can do it, it probably does become valuable to specialize a small model for that task only.
I had Aidan on from Cohere the other day, and he said that it is harder and harder to see visible gains in models, given now the incredible performance and knowledge of them. And so before, you used to be able to kind of take anyone off the street and they'd be smarter than the models. But now, actually, the models have got so smart, it's kind of harder and harder to distinguish.
And it's almost getting to that kind of 92% versus 94%.
I think that actually has much more to do with our benchmarks and the way people typically are used to using these models than the models themselves. If you look at some of the hardest problems that models sort of face, we are still seeing gains to larger models of different techniques, things like this. Part of the problem here actually is sort of these models are a victim of their own success.
People have started to use these very regularly in their daily lives and they probably have a suite of questions that they ask these models. You know, when they first interact with a model, they'll probably ask it to write a biography of history of your school or a biography of yourself or something like this, but you have a suite of questions you sort of ask these models.
And on a lot of these pre-formatted questions that you know models already kind of do well on, the newer models don't do notably better, right? So, if I say, write a history of Carnegie Mellon University, Lama's 7 billion can do that just fine, right? Or 8 billion now can do that just fine, right? There's no need. I mean, maybe it'll be a little bit better with the largest closed source models, but
These aren't the kind of questions that are relevant. The domain I use models for most probably is coding and also doing things like transcribing lectures and stuff like this. On those tasks, I am absolutely not seeing plateauing gains. The latest models, they are notably better than the previous iteration and just make my life easier.
Let me move up to sort of higher and higher levels of abstraction when I give them instructions, when I interact with them, and when I work with them. So This perception has more to do with people's limited imagination of what they can do with these models and less to do with the models themselves. But that will evolve over time.
People will start figuring out you can use them for better and better things.
One thing that I struggle when I look at model ecosystems is just the commoditization of models. I remember a year ago, 18 months ago, it was so expensive, so hard, there were so few players.
Now that there's so many, the commoditization, the reduction in cost, how do you expect this model landscape to play out given the commoditization being really one of the fastest commoditizing technologies we seem to have seen in years?
I think that it's been evolving so quickly between recent releases of open source models, continued progress in the closed source models. There was also this proliferation early on of a lot of open source models that none were better than the other, and they just involved a lot of training for companies, for lack of a better word, to just demonstrate that they could do it too.
It's not clear that's a valuable thing. Why would you want to train your own language model from scratch if there are very good open source ones now? Will that continue? Maybe, maybe not. I think there will be most likely consolidation, but I'm not quite sure how it will play out.
What do you think the model companies that do survive and win, what decisions do you think they'll make? When you said about the proliferation of operating systems and only a few survive, what decisions do you think the model companies that survive and thrive will make to survive and thrive?
I do think that there are a lot of companies that are right now thinking about training their own models and things like this. And it's just sort of the default that, of course, you would do this, that this won't be an economically viable thing to do in the future. And so it won't happen anymore.
We've mentioned data, we've mentioned models. The third kind of pillar is something you've mentioned quite a few times, which is compute. And people are saying now, you know, we've got to the stage of diminishing returns, more compute isn't leading to an aligned level of performance in models. We've really reached this kind of diminishing returns bottleneck.
To what extent is that true, or do we have a lot more room to run and throw in compute?
I'm not really sure what the rationale is for saying that we've plateaued in the compute sense. Most scaling laws that I've seen certainly suggest it can keep going. It's more expensive. You could argue that it's by far just scaling may not be the most efficient way. to achieve better results, and I actually think that's very likely true.
There are other better ways, you could argue, to achieve the same level of improvement than compute, but compute still does seem to be both a major factor and b still seems to improve things. It's more a calculus about also the monetary trade-offs of how much models will cost at inference time and how much they cost to train and all this kind of stuff.
These are much more, I would say, kind of becoming more practical concerns than a concern about the actual limits of scaling.
To what extent do you think the corporations that we mentioned are chasing AGI, super intelligence, versus making amazing products and leveraging AI to make them and make more money?
This question is, I think, super interesting. And those are not actually mutually exclusive, to be clear. One thing I'll also say is that the term AGI is thrown around a whole lot. I define AGI as a system that acts functionally equivalent to a close collaborator of yours over the course of about a year-long project.
So this is something that, you know, you would value as much as a close collaborator, you know, a student of mine or a colleague of mine over working on a project for a year. Let's think about me. AGI would be a system that could automate everything that I do for the most part over a year. That's a pretty high bar.
I am massively uncertain as to when this will happen, but a massive shift that I've undergone is I think this will probably happen in my lifetime. I think the answer to AGI has always been in academia, not in my lifetime. And the timeframe I give this right now is I think this is between four and 50 years or something like this, right? Which really captures my massive uncertainty.
But I have a hard time dismissing it also, given the rate of progress and the things that I sort of see evolving here. We have to take that possibility very seriously.
This is not the same with all new technology introductions to society. There is a gradual curve and there is employment displacement. There is societal upheaval. And that is a natural cycle with technology.
I actually also agree with you that we will adapt to it. I don't want to downplay the extent of transformation that might be necessary here. But I also think the companies that say survive and thrive and become dominant in this new world, the ones that succeed best will not be the ones that fire all their workers to have an AI that does the exact same thing as their old workers.
They'll be the ones that understand, okay, what's changing and what are the things that people can best do now in terms of steering these systems, in terms of sort of providing the overall guidance and framework about where we want to go with with all this intelligence.
The companies that survive, I think, will be the ones that best leverage their workforce to make the best use of this new technology.
Do you think the current providers of models in particular give a particularly good on-ramp to consumers for how to leverage their technologies best?
This is actually a very nuanced question. Do we have AI products that are able to be maximally used by workforces? The answer to this right now is no. Clearly, there is a gap between what people could use these things for and what they're using them for right now.
For large enterprises, a big concern is actually just the mobility or transferability of their data. They want everything on-prem. There's a big unwillingness to have anything trained on their data. Do you think we will see AI bring back a movement from large enterprises away from the cloud back to on-prem?
I mean, I find this kind of interesting in a way because enterprises are all very happy to put their data in the cloud. They all use cloud services to store their data. But then, oh, train on this there? No, no, no. Can't do that. I think a lot of it comes, honestly, from kind of a misunderstanding about how this process works.
Also, frankly speaking, I think it has to do with the fact that if you think about the model of just taking all your internal data and dumping it into a large language model, this is not tenable. You can't do this for a number of reasons. The most obvious one being the data has access rights, right? Not everyone gets access to all the data.
And the default mode of language models is that if you train on some data, you can probably get it back out of the system if you want to enough. And so this doesn't work with the sort of the access controls people have in traditional data. I think these are kind of the concerns. Now, to be clear, there are very easy ways around this, right?
So this is probably why RAG-based systems are so common here and probably will remain, even with the advent of fine-tuning availability, they're going to remain a useful paradigm. RAG is, for those that maybe haven't heard the term, it's retrieval augmented generation. It basically means that you just
You go out and fetch the data you can access, that you have access rights to, that is relevant to your question. You inject it all into the context of the model, and then you answer the question based upon this data here too. These RAG-based techniques are going to remain popular precisely because they respect normal data access procedures.
I sort of feel like a lot of this hesitancy actually comes from a fundamental misunderstanding of how these models are working. People think that if you have ChatGPT answer a question about any of your data, that data is somehow being trained upon and merged into the model, whether it's an API call or whether it's a RAG-based call or anything else. And it's just not true.
That's not how these models actually work. These models are trained once on a very large collection of data. And if you use some of the things like API access and stuff like that, your data's not going to be trained. The model will not be retrained on that. And even if it was, That is not the same thing. The fact that a model can answer your question does not mean the model is training on it.
This is honestly just very simple levels of misunderstanding, I think, that a lot of people have a very hard time getting over. And I still see these misconceptions when I talk with companies. So it's not like we've done, in some sense, maybe a very bad job of marketing because we just don't really always, people don't really understand at some level, sort of, this is not...
in certain use cases, any riskier than just having your data in the cloud to begin with, which all of them typically do. They've all moved that way. So I think this will just happen naturally with progression of time.
What do you think are the other biggest misconceptions that people have towards AGI? There's so many. I mean, I didn't know people actually knew them. But what are others which really frustrate you?
The thing that frustrates me most, honestly speaking, is the degree of certainty that some people have about whether we will definitely get there very, very soon or even more on the flip side, that there's absolutely no way that we will ever achieve AGI with these current models because of X, Y, Z, right? This does actually kind of start to irk me a little bit.
Because I personally, even as a product of the AI winter skepticism, I see what's happening in these models. And I am amazed by it. And the people that have been sort of ringing this bell for a while and saying, look, this is coming. They've, in many cases, in my view, been proven right. And I've updated my sort of posterior beliefs based upon the evidence I've seen.
And so what irks me the most about a lot of people's philosophy of AGI is that, to a certain extent, how little it seems like observable evidence has changed their beliefs one iota. They had certain beliefs about what it would take to get to general AI, or maybe that AI was impossible by definition, or AGI was impossible by definition. And they kind of maintained those beliefs, in my view,
in the face of overwhelming evidence, at least pointing to contrary outcomes.
Can I ask you, you know, a big concern for me actually is misinformation. Yes. It's deep fakes. It's the creation of malicious cyber attacks. I don't think we spend enough time talking about this. When you think about reality underlying practical dangers, what most concerns you if those are some that concern me?
Yeah, so I have a huge number of concerns here and sort of different tiers of concerns, I would say. Let's talk about misinformation, deep fakes, in general, kind of using these tools to proliferate different kinds of misinformation. This is a massive concern, of course, and I am deeply, deeply worried about this.
But the net result of this outcome is not going to be that people start to believe everything that they see in misinformation. The real negative outcome is that people are not going to believe anything that they see anymore, right? So
arguably, we are already well along this way, or well along this path already, where people basically don't believe anything that they read or that they see or anything else that doesn't already conform to their current beliefs. It didn't even need AI to get there. But AI is absolutely an accelerant for this process. What I will say, though, this is not a new phenomenon.
This is actually the human condition as we evolved. It is a relatively new phenomenon that we have a record of objective fact in the world. I mean, things like video didn't exist more than 100 years ago. Humans evolved at a time during an environment where all we could do was trust our close associates. That's how we believed things.
It's in some ways, we see it as tragic right now that we are maybe no longer in a world where we have a record of objective truth. But in another sense, maybe we're just getting back to kind of the world that we used to live in, where all we could do was trust our close associates about what we believe about the world.
Does that not lead to a reduction in the advancement of human knowledge, though, if we only trust the people around us who we've known for years when we see them in person, not even when they send us something?
Yeah. I mean, so obviously there are massive negative externalities, but we did evolve knowledge very well at a time before video, right? Before videos existed, we still made scientific progress. So there will be groups that decide that the certain bodies of scientific knowledge are valuable and they will advance them.
even in light of large other portions of the population, which have existed throughout all of history too, that don't value those scientific advances or think differently about the nature of scientific advances. We are already in this world, right? This is the world we already live in.
I think it is definitely an accelerant and a shame that this sort of puts us more toward the camp of failing to have an objective reality. But humans, it's arguably our natural state is to not agree on the nature of objective reality.
But I think to me, this is why you see the increasing value of existing media brands, because people place validity and trust in the content that they produce. So you trust the New York Times tweet where it shows something, some random account which has a picture, don't know.
You could argue that it's going to be a whole group of people that don't believe anything the New York Times says. There is already that group, by the way. There's plenty of countries where they would not believe anything that's published in the New York Times, right? So we're already there to a certain extent.
And I think, yes, we will need to rely arguably more on groups, but also sort of their associated belief structures about this. But this is the human condition to a certain extent. Not to get too philosophical here, but this is how we've always kind of had to be.
Video is a short blip where we sort of think that there's some objective evidence for 100 years of our history, and that's going to be now. That's no longer true pretty soon.
When you think about AI safety, though, then, should the platforms themselves be the arbiters of justice of what's right and what's not right? You know, Twitter, Facebook, Reddit. And are they the ones that say, no, this is not allowed content?
There are some things I believe that should not be shared on social media. And by the way, everyone else agrees with this too, right? There's obviously content that is outright considered illegal that you cannot post to social media. Everyone agrees on this.
Everyone also agrees that, well, not everyone, but a lot of people also agree that in general, there should not be a requirement to conform to certain ideologies and opinions if you want to express yourself on social media.
And so there's obviously a middle ground you have to and you have a total line here and you have to adapt to the reality of the situation on the ground and kind of go from there in many ways.
And this is maybe what I was pointing out before is that AI, when it comes to things like misinformation, it did not invent misinformation, AI, it can argue there was misinformation and propaganda and this stuff long before there was AI. You can argue it's an accelerant for everything, for a lot of things that we have, right? But it does not invent these things.
And my hope, at least, is that a lot of our existing social and economic and governmental structures can continue to provide the same guidance they provided for our current take on moderation and things like this, even in an AI world.
How do you respond as a government organization today where you are supposed to set regulation, supposed to set policy, and you are dealing with rag, flops, architect, transformer architecture? All of these technical words and architectural information that they have no idea what it means. My question is, are governments structurally set up to regulate AI effectively?
I hold two beliefs at the same time. Like a lot of new technologies, there's absolutely a role for regulation and for governments to provide frameworks for ensuring that new technologies do benefit the world. This is why we form governments to a certain extent. In that umbrella, I believe there is absolutely the need to better understand how and where we can regulate AI as a technology.
I also, though, think that maybe to your point of the examples you were given, a lot of the details about how those regulations sometimes evolve can be a bit misguided or miss the point.
Or somehow, when I read them, basically, they're going to become dated in a matter of months, because they're dealing with things and they're approaching the problem from a way that doesn't really match the nature of how these systems are really developed in practice. I think that it is much easier. We have a much better handle on regulating the downstream uses of AI.
Like when it comes to misinformation, we already have laws that deal with sort of libel and things like this.
In many cases, because AI is acting as an accelerator, there are situations in which I think that existing laws, maybe with a slight tweaking to deal with the velocity and the volume that AI is capable of producing, can suffice to regulate many of what we consider the harmful use cases of AI. But at the same time, I don't think that's efficient either.
Of course, there are going to be ways in which technologies, especially technologies as powerful as this one, we have to think about ways in which we can regulate it. I don't know what that looks like. I think it's extremely hard because it changes incredibly rapidly.
Speaking of kind of the safekeeping models, a terrible interview that I am kind of jumping between so many different topics, but I do want to discuss the hierarchy of safety concerns that you have. Because I mentioned mine. How would you categorize yours?
Sure. The biggest concern I have right now in AI safety, which I think leads to a lot of negative downstream effects, is that right now, the AI models that we have, for lack of a better phrasing, are not able to reliably follow specifications. And what I mean by this is that these models are tuned to follow instructions.
You can give them some instructions as a developer, but then if a user types something, they can follow those instructions instead, right? We've all seen this. This goes by a lot of names, prompt injection. Sometimes, depending on what you're getting out, this is called things like jailbreaking and things like this.
The core point is we have a very hard time enforcing rules about what these models can produce, right? So oftentimes we say, you know, models are trained right now just to not do things. I use a common example of things like hot wiring, hot wiring a car and I'll have demos I give, right? So models are trained. If you ask the model, you know, most commercial models, how do I hotwire a car?
They'll say, I can't do this. It's very easy through a a number of means to basically manipulate these models and convince them that they really should tell you how to hotwire a car because you know, you're in desperate need of, you've locked yourself out and it's an emergency and if you don't get in your car, this is very different from how we're used to programs acting, right?
We are used to computer programs doing what they're told, nothing more and nothing less. And these models don't always do what they're told, sometimes do too much of what they're told and do way more than what they're told also some other times. And so we are very unused to thinking about computer software, rather, like these models.
And what that means is, and to be honest, I don't really care if models tell me how to hotwire a car. I just don't. It doesn't matter, right? There's instructions on the internet on how to hotwire a car. They're not really revealing anything that sensitive. However...
As we start to integrate these models into larger systems, as we start to have agents that go out and do things, that parse the internet and go out and do things, if all of a sudden they're running their model, parsing untrusted third-party data, that data can essentially gain control of those models. To a certain extent.
And this is from a sort of cybersecurity standpoint, not the normal cybersecurity, but sort of from a concept of cybersecurity. This is sort of like these models have a buffer overflow in all of them that we know about. And most importantly, that we don't know how to patch and fix. We don't know how to fix this yet with models. To be clear, I think we can make a lot of progress.
We are making progress. But this is a real concern about models right now. And the negative effects in a domain like a chatbot are maybe not that concerning. But as you start having much more complex LLM systems, this starts becoming much more concerning.
What I will also say is that, and this is maybe the reason why I placed this concern first, is that I think this fact is something we need to figure out, or kind of all the other downstream concerns that we have about these models get much, much worse. So let me just take an example. Oftentimes, I'm touching a lot of points here I know too, but I think I'll wrap it up soon.
Oftentimes, people talk about risks like bio risks or cyber attack risks and stuff like this. I'm actually, to your point, I'm very concerned about cyber risks in particular. I think this is essentially already solved in many cases by these models. They can already solve and analyze code to find vulnerabilities. This is extremely concerning.
The way we think about fixing this normally is, you know, we would have certain models, models that we release. We would say, you know, don't use your model ability that you have inside of you to sort of create obvious cyber attacks against certain infrastructure and stuff like this, right? Don't do that. But we can't make them follow that instruction, right?
Someone either with access to a model itself, certainly, but even with access sometimes to a closed source model just can have the ability to jailbreak these things and oftentimes get access to these things, right? To be very clear, we are making immense progress in solving this problem of sort of preventing jailbreaks, kind of avoiding making sure models follow a spec.
But until we can solve this problem, it's very hard to say, you know, all the other dangerous capabilities that AI could sort of demonstrate become much, much more concerning. And so this is kind of a multiplier effect on everything else bad these models can do, which is why I'm so concerned about it right now.
And the multiplier effects, the subsequent elements that become heightened because of this are like terrorist attacks, are fraud cases, are... So, right.
So that's sort of a good lead in, right? Because if jailbreaks and sort of manipulation of models is the attack vector, what is the payoff? What are the things we can do? And here, what we're trying to do really is we're trying to assess the core harmful capabilities of models, right? And people have thought a lot about this, right?
People think about things like creating chemical weapons, creating biological weapons, creating cyber attacks. Personally, I think cyber attacks are a much more clear and present threat than, for example, bio threats and things like this. At the same time, I don't want to dismiss any of these concerns, right?
I think people have looked at this much, much more than myself and are very concerned about these things. So I want to treat this with the respect that honestly it deserves because these are sort of massive problems. There are a lot of potential harms of AI models. Some are associated primarily with scale and things like this, like the misinformation you mentioned.
But some are just, there are capabilities that we think these models might enable where they would lower the bar so much for some bad things, like, say, creating a zero-day exploit that takes down software over half the world. The concern is that, not that they can do this sort of autonomously, maybe initially, but
but that they can lower the bar so far in the skill level required to create these things that effectively it puts them in the hands of a huge number of bad actors. And the same is true for things like biological risk or chemical risk or other things like this. And these concerns have to be taken seriously.
And they have to be things that we really do consider as genuine possibilities if we start putting into everyone's hand the ability to create
really sort of harmful artifacts alex wang at scale said a brilliant line on the show he said that essentially we have a technology now that is more potentially dangerous and impactful than nuclear weapons if that is the case or even partially the case or even potentially the case is there any world in which it should be open
Two issues there. One is AI as dangerous as nuclear weapons, and what does this imply about the open release of certain models? So I'll make two points on this. I think the nuclear weapon analogy is actually not a great one, because nuclear weapons have one purpose, which is to destroy things.
Maybe a better analogy is sort of nuclear technology period, because it has the ability to create nuclear weapons, but it also has the ability to do things like provide power, non-CO2 emitting power to potentially a huge number of people, right? A lot of people are currently making a bet on nuclear as the way we create carbon-free energy.
But I think the analogy of nuclear weapons in particular is often overstated precisely because AI has many good uses. Nuclear weapons, arguably, they do one thing, and it's not considered a good use, right? So there's a very different kind of technology there.
But let me get to your second point now, which is the sort of the open model debate, which is also one that frequently is played out in kind of discussions on AI safety. I should start off by saying I'm a fan of open source models in a general sense.
So I want to start by saying that because honestly speaking, open source release of models and I really say open weight because oftentimes these are not actually open source traditional way. They're actually much more like closed source executables. They just you can run them on your own on your own computer. Open weight models. have advanced my ability to study these systems.
They've been the primary tool by which we conduct research in academia and beyond, and they are becoming, I would argue, a critical part of the overall ecosystem of AI right now, number one. Number two,
If you look at the current best models that there are right now, so things like GPT-4, Claude 3.5, Gemini, things like this, I would not currently be all that nervous about having an open source model that was as capable of these in terms of the catastrophic effects of it. Because these models actually aren't by themselves. We have a good handle on them, right?
We sort of know what they're capable of. Arguably, we're already here because Lama 3, 405 billion is pretty close. I don't think it's quite at that level yet, but it's getting there. And, you know, this release has not yet caused some catastrophic event. Because the reality is these models, they still have a ways to go.
Right now, to a certain extent, I think things are okay with open weight release of the models. However, there will come a time when a certain capability, a certain ability of these models reaches the point that should give us pause when it comes to just turning these things over to whoever and everything. what, however they want to use them.
And I do think this, there are certain levels of capabilities that you could see that are within kind of eyesight of our current development. That if I was sort of just to ask the question, no, should, should we give this to everyone, not just to use, but to use and tune and specialize however they want.
And I would just sort of say, I think there will be a point where I get uncomfortable with that. What is that point? So if you think about a model that really could analyze any code base or even any binary executable or website or JavaScript or anything like this and immediately find a vulnerability that it could exploit to take down a large portion of the internet or a large portion of software.
If this was demonstrated as a capability of a model, I would have a very hard time saying, of course, yeah, let's just release it. You know, there's no problem because we'll use it for good. That's dual use, so we'll use it for good purposes. We all know that patching software is much harder than finding exploits in software. Patching all software is much harder than finding exploits in software.
Yes, there's dual use. You can use it to secure software better, but that takes time. It's hard. I don't think we should just immediately snap to release a model that could find a vulnerability in literally any code that's out there in the world. And so I wouldn't want that to be released open-weight for anyone to use.
Now, I take some solace in the current situation we find ourselves in, which basically in the current situation we find ourselves in, at least for now, there's a constant stream of closed models that are released sometime before an equivalently capable open-weight model. And I think this is actually a very good thing.
Because my hope would be that and we've sort of found ourselves here by accident. It didn't have to be like this. I know some companies are pushing to open source more powerful models than we have ever had before right at the outset. That makes me a little nervous. But right now we're not in that world.
We're in a world where the most capable models, the first releases of them of a certain capability typically comes from closed source models. I think this is a good thing.
I think it gives us some time to essentially come to terms and understand the capabilities of these models in a more controlled environment such that we can reach a level of comfort to say, maybe not full comfort, but at least a level of comfort to say, yes, it's probably okay if we release this, a similar model open source.
And I hope, my sincere hope would be that if one of these models does really demonstrate the ability to create an exploit for any executable code or compiled code or anything else instantly, and we see that in the closed source model first, we would think a little bit about whether we really want to release this model, an equivalent model, open weight, and just for anyone to use.
Is there anything I have not asked on AI safety that I should have asked?
I do think that the more far-fetched scenarios about sort of agentic AGI systems that start sort of intentionally acting harmful against humans, the rogue AI that decides it wants to wipe out humanity and goes about planning on how to do this.
These more, I would say, what seem to me, and I'll be honest here, far-flung sci-fi-ish scenarios here, these are often the debates we have when it comes to AI safety. I want to say two things about this. The first is that I think the vast majority of AI safety should not be about these topics.
The vast majority should be about quite practical concerns we have on making systems safer, like the kind that I've talked with you about so far. There are already massive
safety considerations and risks that are present in current systems and would certainly be present even in slightly more capable systems, irrespective and regardless of the timeframes associated with AGI and certainly the timeframes associated with rogue intelligent AI systems. However, I also don't want to dismiss this entirely.
The way I would put it is I am glad people are thinking about these problems, I'm glad people are thinking about the capabilities and even what I consider far-flung scenarios. They are good things to think about as, by the way, are much more immediate harms of AI systems like misinformation, like misuse of these things.
What far-flung scenario do you think is most good to think about? Because most people just go robots, killing jobs, killing humans, ultimately post-killing our jobs. Yeah.
I think killing jobs is much more immediate of a concern than killing humans. An example I often use here to kind of try to bring a little bit of these two sides, the AI taking over the world, killing us all, and kind of the more skeptical minded academic folks, we'll say.
I see a path right now to a world in which, you know, in a few years from now, we start integrating AI models into more and more of our software. We start building it up more and more. We sort of make these things a little bit more autonomous in their actions.
We start just naturally, because software does everything for us, we start naturally kind of infusing this into all software we have, including software that handles things like critical infrastructure, stuff that controls the power grid, things like this, right?
And now all of a sudden, you have these agents that are sort of, you know, taking an active, playing an active role in doing things like controlling power grids. This leads to the possibility of, even in my view, sort of massive correlated failures that could do things like bring down power, electricity in a way that we can't restore it easily in for a large portion of the country.
And I think it's honestly not again, if we go down the wrong path, this is definitely not that impossible to imagine right now in this world where the power has been shut off, you know, we can debate and decides can debate about whether this was a bug in the system. And we should never have installed LLMs here in the first place.
Or we could debate whether this was actually the rogue AI taking over and deciding to shut off the power so it could kill all humanity. But who cares? The power is still off. This is still a catastrophic event for the country. And so we have to have a plan for how to sort of think about events like this happening. This is an example I come to often.
To a certain extent, it doesn't matter whether the AI is intentionally doing something in an evil fashion while deceiving humans, or whether this is a bug and a flaw in the system. The end effects are the same in some cases. And so we need to desperately take kind of put in structures in place that prevent these things from being possible.
Or we just appreciate that they had CrowdStrike.
Well, exactly. So yeah, we are all very familiar right now with the downsides of correlated failure, right? And imagine if that was also true of all the SCADA systems that were operating power, the power grid right now, which, you know, not impossible to believe. And the problem is that these systems, because we don't understand, really, I mean, and we don't, we don't understand them, right?
We do not understand how these things work internally, the possible correlated failures, the possible attack factors, all these sorts of things. We don't understand it.
And because of this, we need to think very carefully about how we deploy these systems, how we do consider safety concerns, especially when it comes to things like critical infrastructure that I think are extremely pressing concerns. And yes, things like bio-risk, again, these I work much less on, but pressing concerns. And you don't have to believe in super intelligent systems.
evil robots, in order to have these as pressing concerns, AI safety is a concern right now. And we all need to come to grips with the fact that it's a concern right now and start solving the problems right now.
The astonishing thing is, I don't know if you remember banking, but password tests were like, my voice is my password. I really hope yours isn't right now because 11 Labs is doing pretty great things with my voice.
Yeah, it's really wild. The current things that we have already upend a massive amount of sort of the systems we've built in place, and they will continue to be upended more and more from evolving AI technology. And these are real concerns that we have to come to terms with.
Are you optimistic about this future we're moving into? And do you want your children to speak more to LLMs and models than they do to humans?
I would classify myself as an optimist when it comes to AI. I already enjoy these tools. I'm excited about the potential things we can do with these tools. Yes, even up to AGI. And I use the word tool here, not pejoratively. Hopefully, AGI is a tool, right? Hopefully, AGI is a system that we still deploy to our ends to achieve our ends. I can't help but be excited about these things.
This is the culmination of a lot of the work that we in the field have been doing. It's kind of coming to fruition in a lot of ways in a way that is directly sort of beneficial for a lot of the things that I do. So I want to have these tools and maybe this gets to the clear point here. I want to develop and improve safety of these tools and because I want to use them.
It's not that we have some moral imperative that we have to develop these tools. I mean, maybe there are, or we have to develop AI and AGI. Maybe that's true, but that's not what motivates me to develop them, right? I want to develop them because I want to use them and I want to be able to have them to reach that point. They have to be safe, right? It's a condition, it's a necessary condition.
And that's why I work on building and improving the safety of AI systems.
I could talk to you all day. I do want to move into a quick fire. I say a short statement. You give me your immediate thoughts. Does that sound okay? Okay. What did you believe about models that you later changed your mind on?
I, for a lot of my career, was thinking that model architectures really mattered. And by having clever, complex architectures and sub-modules inside architectures, that was the route to better AI systems. For the most part, I don't believe this as much anymore. I think basically models don't matter. Architectures don't matter. And that applies to transformers too.
I think, you know, anything could kind of work in their stead if we just spend enough time on it. And so I think that to a large extent, we're kind of post architecture in a lot of our AI work.
What a breakthrough snap that is. What did you believe about data that you later changed your mind on?
Kind of on the contrary, I thought that data was sort of, you know, data had to be highly curated to be valuable. And the value in data came essentially from very manual labeling of this data and human intensive curation.
The big, amazing insight of current AI is that we can, to a large extent, just suck up data that exists out there on the internet, train models based upon that, and get amazing things to come out of it. Not to say there's no value in curation. Of course, there's elements of this, but to a very large extent, this is kind of on the old paradigm of unsupervised learning. That's absolutely incredible.
How does joining the OpenAI board look? Does Sam just call you up and go, hey, love the whiteboard, fancy coming on our board?
Um, I got actually the, the, the, the day before I started as department head, I got an email from, from, from Brett, the chair of the board, just saying, Hey, do you want to talk about maybe joining the open AI board? Uh, so I figured, you know, I was already embarking on one massive career change. So why not, why not double down and just do, you know, do two at the same time.
But basically I, I, I started having some conversations with him and the rest of the board. I got very excited about the potential sort of provide my perspectives on AI and AI safety to the board and things went from there.
What are the roles and responsibilities? Do they set them out like four board meetings a year and, you know, a biscuit and a coffee in between?
There are four board meetings a year, yes. But I think I'm being brought on the board as an expert in AI and AI safety. I am excited to provide my perspective and expertise specifically on AI to the rest of the board.
Do you believe the statement that China's two years behind the US in terms of AI progression?
There is absolutely some element here of kind of a race between different countries for AI dominance. I actually will take a different stance on this and say that I think there are certain things like, for example, AI safety, where we very much need to work as a world to help set standards and help better the future of everyone here.
Because yes, certain things can be done by countries, capabilities can maybe advance more by countries. Safety is something that's inherently global. systems.
Final one for you. What is the most common question you're asked that you don't think you should be asked?
The most common one has to do, I would say, with questions that put an overemphasis on the architectures involved in AI systems. So, you know, this notion that somehow the transformer was the thing that made all AI possible. I'm often asked questions like, you know, what comes after the transformer and things like that.
And the reality is, as I said before, and which probably I know makes for a good soundbite architect, we are arguably in a post architecture phase, they don't they don't really matter, we could do what we're currently doing with a whole lot of architectures right now.
And I hope that I can steer the conversation to more of one where we consider these models not in terms of their particular structure, because it's somewhat irrelevant when it comes to capabilities. And we think about these models more in terms of the data that goes into them, and the capabilities they produce downstream.
As you can tell from my meandering conversation, I've so enjoyed this. I'm so glad you didn't have too much time with the schedule, otherwise I would have been screwed. But thank you so much for being so brilliant.
Great. Well, thank you very much for inviting me.
That was so much fun to have Zika on the show. And if you want to watch the full interview, you can find it on YouTube by searching for 20VC. That's 20VC. But before we leave you today, when a promising startup files for an IPO or a venture capital firm loses its marquee partner, being the first to know gives you an advantage and time to plan your strategic response.
Chances are the information reported it first. The information is the trusted source for that important first look at actionable news across technology and finance, driving decisions with breaking stories, proprietary data tools and a spotlight on industry trends.
With a subscription, you will join an elite community that includes leaders from the top VC firms, CEOs from Fortune 500 companies and esteemed banking and investment professionals. In addition to must-read journalism in your inbox every day, you'll engage with fellow leaders in their active discussions or in person at exclusive events.
Learn more and access a special offer for 20VC's listeners at www.theinformation.com slash deals slash 20VC. And speaking of incredible products that allows your team to do more, we need to talk about SecureFrame. SecureFrame provides incredible levels of trust to your customers through automation.
SecureFrame empowers businesses to build trust with customers by simplifying information security and compliance through AI and automation. Thousands of fast-growing businesses including NASDAQ, AngelList, Doodle, and Coda trust SecureFrame to expedite their compliance journey for global security and privacy standards such as SOC 2, ISO 2701, HIPAA, GDPR, and more.
backed by top-tier investors and corporations such as Google, Kleiner Perkins. The company is among the Forbes list of top 100 startup employers for 2023 and Business Insider's list of the 34 most promising AI startups of 2023. Learn more today at secureframe.com. It really is a must. And finally, a company is nothing without its people.
The global law firm built around startups and venture capital. Since forming the first venture fund in Silicon Valley, Cooley has formed more venture capital funds than any other law firm in the world, with 60 plus years working with VCs. They help VCs form and manage funds, make investments, and handle the myriad issues that arise through a fund's lifetime.
We use them at 20VC and have loved working with their teams in the US, London and Asia over the last few years. So to learn more about the number one most active law firm representing VC-backed companies going public, head over to Cooley.com and also CooleyGo.com, Cooley's award-winning free legal resource for entrepreneurs.
As always, I so appreciate all your support and stay tuned for an incredible episode coming this Wednesday.